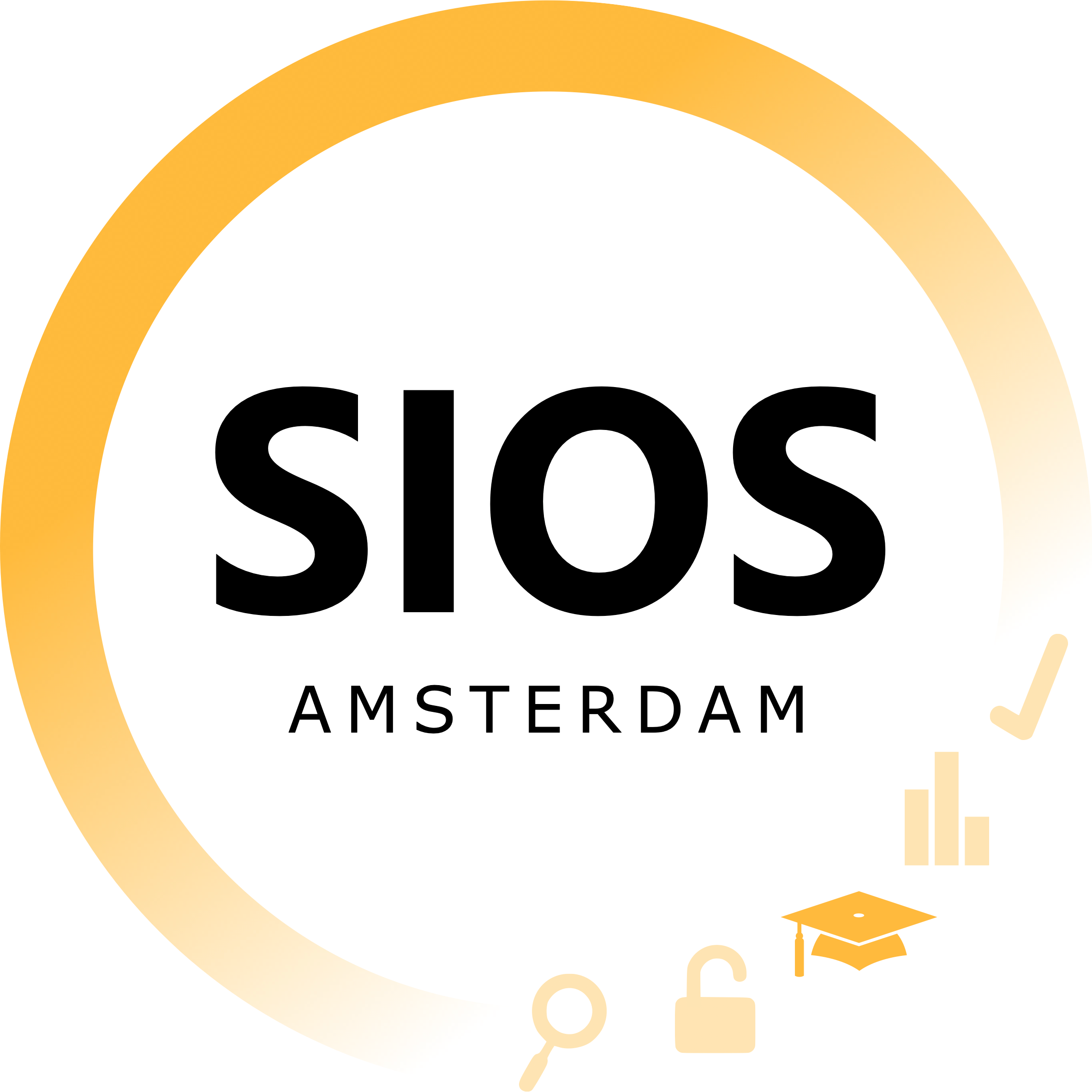
The Dunning-Kruger effect, introduced by two Ivy League professors in 1999, states that poorly performing people tend to overestimate their skills while well-performing people underestimate them. It is one of the first cognitive biases that undergraduate psychology students are taught, and as the initial finding is based on a series of logical reasoning, grammar, and social skill assessments, its premise extends well into classrooms. During the first months of our degrees, we are continuously reminded about our biased nature of reasoning, especially when it comes to the body of knowledge we hold over a topic – we are encouraged to be critical about our estimation of our own competence, irrespective of whether it stems from overconfidence or insecurity. Yet many of us fall prey to this alleged phenomenon as we leave the exam hall mourning all the wasted time and effort, sure about having failed the test but having passed it after all. Even before first hearing a professor lecture about the effect, we are all more than familiar with it: everyone knows someone without a relevant degree who at a family gathering claims to know the political solutions to the biggest economic issues or someone who does not believe that they are good enough to participate in an arts or sports competition. That is why to many the following will come as a surprise: a few years ago, the Dunning-Kruger effect was debunked. On top of it being easily explainable with the combination of the statistical phenomena regression toward the mean and the better-than-average effect, it seems that it is merely an artefact; an autocorrelation.
Nothing can put down a psychology student already questioning their career choice like this. Are all cognitive biases we learnt about utter nonsense? We knew that correlation does not imply causality, but not that sometimes even the correlation as itself cannot be trusted.
A look into the methodology of the 1999 study with more developed statistical knowledge reveals that their data analysis procedure lacks sufficient control over measurement error. A replication attempt by Gignac and Zajenkowski (2020) explains this in a simple manner: appropriate statistical tests were not available during the conduction of the initial study, hence leading to an overestimated effect. When the same data is analysed with fitting tests such as the Glejser test of heteroscedasticity and nonlinear regression, the p-value jumps above the threshold of significance. Later, in April 2022, political economist Blair Fix wrote a piece in which he shows the effect to be due to autocorrelation, the correlation of a variable with itself. In it, he demonstrates how Dunning and Kruger rightfully plotted the average percentile of the actual test score, x, with the average percentile of perceived ability, y, but then asked us to look at the difference between those lines, x – y. This leads to us implicitly comparing x – y to x, which gives rise to an autocorrelation. As many of the biggest and prevailing findings on the psychology of humans were found during the same era, we cannot help but wonder whether any of them would stand a chance in today’s world of more resources for replication and better statistical analysis and interpretation skills.
This failure to reproduce the Dunning-Kruger effect is at the core of the replication crisis, an ongoing methodological crisis caused by the difficulty or even pure impossibility to replicate scientific findings. In 2015, led by Professor Brian Nosek, a group of researchers redid 97 studies that were previously published in well-established psychology journals. Surprisingly, they were able to replicate the significance in only 36% of these studies, putting the whole field of psychology under the academic world’s microscope.
“Researchers appear to have too high a degree of flexibility when it comes to data collection and interpretation, suggesting that their hands must be tied beforehand.”
Although in the case of the Dunning-Kruger effect the failure to replicate the findings was due to poor statistical interpretation, this may not be the case for all the other studies that did not stand a chance against the Reproducibility Project. The truth is that in many cases, findings are full-on fabricated or falsified, either by collecting or removing data or trying out different statistical tests until a significant result is obtained. In fact, in an article published by John, Loewenstein, and Prelec (2012), the self-reported questionable research practice (QRP) prevalences were laid out in the table:
- 72% of the respondents admitted collecting data until significant results were obtained
- 39% admitted rounding down p-values
- 42% decided to not report all conditions
- 9% reported having falsified data
Although critizised by Fiedler and Schwarz a few years later, the article raised a fruitful discussion about QRPs and the factors that drive researchers to engage in them. The conversation concludes that researchers appear to have too high a degree of flexibility when it comes to data collection and interpretation, suggesting that their hands must be tied beforehand. This is where the open science framework steps in. By committing to an analysis and reporting plan before the start of the study, the urge for data dredging is minimised. It should be noted, however, that this need for significant results does not appear out of the blue, but that there is substantial pressure from the side of the funders and academic journals for individual researchers to perform in accordance with the hypothesis myopia. In other words, it is easier to get your work published by modifying the results than by reporting non-significance truthfully. (For more insight on the machinery of academic journals, I’d highly recommend checking out a fellow SIOS member’s article for Spiegeloog, From Public Findings to Private Journals.). Luckily, the open science community is already fighting hard against this culture, steering its wheel toward better tolerance of null results. Numerous journals such as Positively Negative and The All Results Journal have provided a platform to publish studies that traditional journals might reject due to non-significance. A great example closer to home is The Psychological Science Accelerator, a project led by the University of Amsterdam lecturer, Dr. Cameron Brick. As a global network of research laboratories it coordinates vast datasets for different research groups that follow the open science framework, and promises to publish the findings, no matter the significance.
“We should not be dispirited by failed replications but instead cherish the advancements they bring about.”
As researchers, we have to accept that mistakes have to happen in order for science to progress. In a true Platonistic fashion, we believe in an absolute truth that exists somewhere, in reality, only our knowledge and biases derail us from the path of finding it out. Thus, we should not be dispirited by failed replications but instead cherish the advancements they bring about. As the psychology students of our time are more and more trained in statistical analysis and interpretation, we can be sure that the future of science is brighter than its past – within the field of psychology, too.
In the end, there is a charming irony to the debunking of the Dunning-Kruger effect. Here are two high-ranking professors from respected universities who for over a decade lectured about the dual burden of unskilled people; the incompetence paired with unawareness of it. It turns out that in this case, it was these academics who were unskilled. Perhaps this should serve as a reminder of our own biased nature of reasoning, and a call for more statistical training and transparent research practices. <<
Student Initiative for Open Science: This Month
This article has been written as part of an ongoing collaborative project with the Student Initiative for Open Science (SIOS). The Amsterdam-based initiative is focused on educating undergraduate- and graduate-level students about good research practices.
Interested in being part of implementing the open science framework into the student community and academic world? Join us!
The Dunning-Kruger effect, introduced by two Ivy League professors in 1999, states that poorly performing people tend to overestimate their skills while well-performing people underestimate them. It is one of the first cognitive biases that undergraduate psychology students are taught, and as the initial finding is based on a series of logical reasoning, grammar, and social skill assessments, its premise extends well into classrooms. During the first months of our degrees, we are continuously reminded about our biased nature of reasoning, especially when it comes to the body of knowledge we hold over a topic – we are encouraged to be critical about our estimation of our own competence, irrespective of whether it stems from overconfidence or insecurity. Yet many of us fall prey to this alleged phenomenon as we leave the exam hall mourning all the wasted time and effort, sure about having failed the test but having passed it after all. Even before first hearing a professor lecture about the effect, we are all more than familiar with it: everyone knows someone without a relevant degree who at a family gathering claims to know the political solutions to the biggest economic issues or someone who does not believe that they are good enough to participate in an arts or sports competition. That is why to many the following will come as a surprise: a few years ago, the Dunning-Kruger effect was debunked. On top of it being easily explainable with the combination of the statistical phenomena regression toward the mean and the better-than-average effect, it seems that it is merely an artefact; an autocorrelation.
Nothing can put down a psychology student already questioning their career choice like this. Are all cognitive biases we learnt about utter nonsense? We knew that correlation does not imply causality, but not that sometimes even the correlation as itself cannot be trusted.
A look into the methodology of the 1999 study with more developed statistical knowledge reveals that their data analysis procedure lacks sufficient control over measurement error. A replication attempt by Gignac and Zajenkowski (2020) explains this in a simple manner: appropriate statistical tests were not available during the conduction of the initial study, hence leading to an overestimated effect. When the same data is analysed with fitting tests such as the Glejser test of heteroscedasticity and nonlinear regression, the p-value jumps above the threshold of significance. Later, in April 2022, political economist Blair Fix wrote a piece in which he shows the effect to be due to autocorrelation, the correlation of a variable with itself. In it, he demonstrates how Dunning and Kruger rightfully plotted the average percentile of the actual test score, x, with the average percentile of perceived ability, y, but then asked us to look at the difference between those lines, x – y. This leads to us implicitly comparing x – y to x, which gives rise to an autocorrelation. As many of the biggest and prevailing findings on the psychology of humans were found during the same era, we cannot help but wonder whether any of them would stand a chance in today’s world of more resources for replication and better statistical analysis and interpretation skills.
This failure to reproduce the Dunning-Kruger effect is at the core of the replication crisis, an ongoing methodological crisis caused by the difficulty or even pure impossibility to replicate scientific findings. In 2015, led by Professor Brian Nosek, a group of researchers redid 97 studies that were previously published in well-established psychology journals. Surprisingly, they were able to replicate the significance in only 36% of these studies, putting the whole field of psychology under the academic world’s microscope.
“Researchers appear to have too high a degree of flexibility when it comes to data collection and interpretation, suggesting that their hands must be tied beforehand.”
Although in the case of the Dunning-Kruger effect the failure to replicate the findings was due to poor statistical interpretation, this may not be the case for all the other studies that did not stand a chance against the Reproducibility Project. The truth is that in many cases, findings are full-on fabricated or falsified, either by collecting or removing data or trying out different statistical tests until a significant result is obtained. In fact, in an article published by John, Loewenstein, and Prelec (2012), the self-reported questionable research practice (QRP) prevalences were laid out in the table:
- 72% of the respondents admitted collecting data until significant results were obtained
- 39% admitted rounding down p-values
- 42% decided to not report all conditions
- 9% reported having falsified data
Although critizised by Fiedler and Schwarz a few years later, the article raised a fruitful discussion about QRPs and the factors that drive researchers to engage in them. The conversation concludes that researchers appear to have too high a degree of flexibility when it comes to data collection and interpretation, suggesting that their hands must be tied beforehand. This is where the open science framework steps in. By committing to an analysis and reporting plan before the start of the study, the urge for data dredging is minimised. It should be noted, however, that this need for significant results does not appear out of the blue, but that there is substantial pressure from the side of the funders and academic journals for individual researchers to perform in accordance with the hypothesis myopia. In other words, it is easier to get your work published by modifying the results than by reporting non-significance truthfully. (For more insight on the machinery of academic journals, I’d highly recommend checking out a fellow SIOS member’s article for Spiegeloog, From Public Findings to Private Journals.). Luckily, the open science community is already fighting hard against this culture, steering its wheel toward better tolerance of null results. Numerous journals such as Positively Negative and The All Results Journal have provided a platform to publish studies that traditional journals might reject due to non-significance. A great example closer to home is The Psychological Science Accelerator, a project led by the University of Amsterdam lecturer, Dr. Cameron Brick. As a global network of research laboratories it coordinates vast datasets for different research groups that follow the open science framework, and promises to publish the findings, no matter the significance.
“We should not be dispirited by failed replications but instead cherish the advancements they bring about.”
As researchers, we have to accept that mistakes have to happen in order for science to progress. In a true Platonistic fashion, we believe in an absolute truth that exists somewhere, in reality, only our knowledge and biases derail us from the path of finding it out. Thus, we should not be dispirited by failed replications but instead cherish the advancements they bring about. As the psychology students of our time are more and more trained in statistical analysis and interpretation, we can be sure that the future of science is brighter than its past – within the field of psychology, too.
In the end, there is a charming irony to the debunking of the Dunning-Kruger effect. Here are two high-ranking professors from respected universities who for over a decade lectured about the dual burden of unskilled people; the incompetence paired with unawareness of it. It turns out that in this case, it was these academics who were unskilled. Perhaps this should serve as a reminder of our own biased nature of reasoning, and a call for more statistical training and transparent research practices. <<
Student Initiative for Open Science: This Month
This article has been written as part of an ongoing collaborative project with the Student Initiative for Open Science (SIOS). The Amsterdam-based initiative is focused on educating undergraduate- and graduate-level students about good research practices.
Interested in being part of implementing the open science framework into the student community and academic world? Join us!