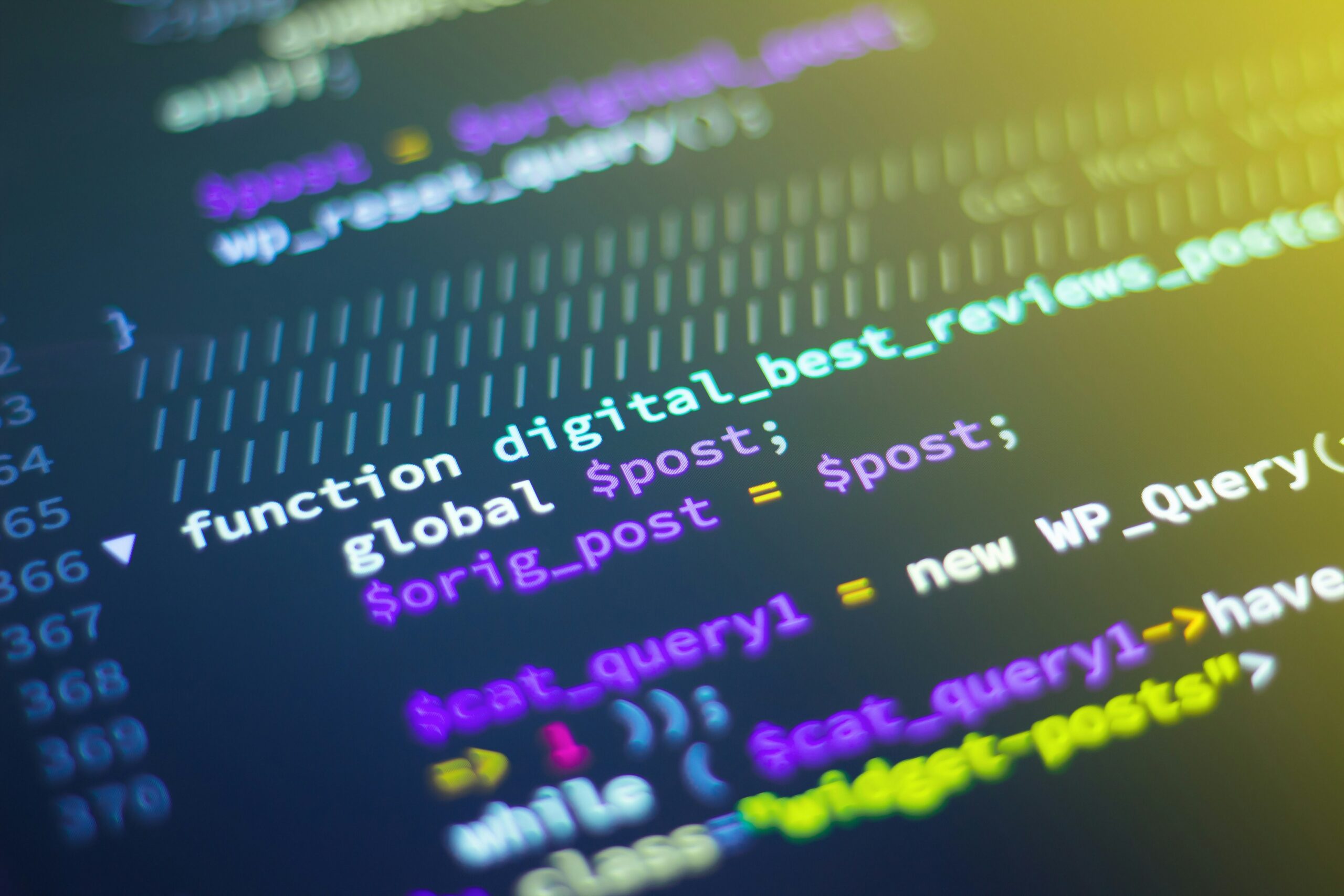
Language defines how we think, communicate, and make sense of the world. In the age of AI, tools like ChatGPT are not just assisting us—they are subtly transforming the way we use language. Large Language Models are slowly reshaping our communication, vocabulary, and even biases in our expression. So, as we increasingly rely on these tools, what should we be aware of?
Language defines how we think, communicate, and make sense of the world. In the age of AI, tools like ChatGPT are not just assisting us—they are subtly transforming the way we use language. Large Language Models are slowly reshaping our communication, vocabulary, and even biases in our expression. So, as we increasingly rely on these tools, what should we be aware of?
Photo by Shahadat Rahman
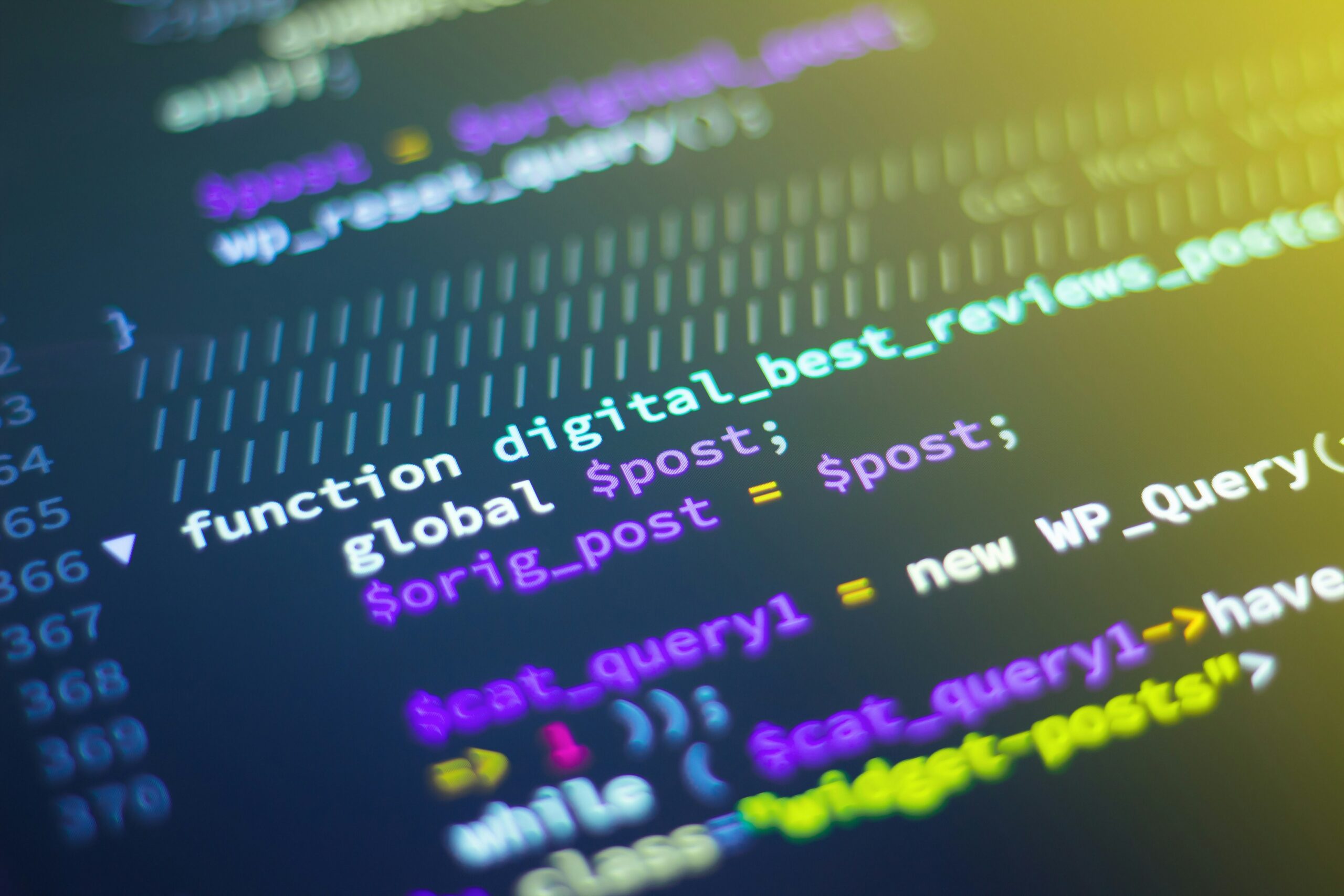
Photo by Shahadat Rahman
No matter the task, assignment or question, ChatGPT has become my new Google. In some way, I cannot even equate it with Google – I would never converse with Google the same way – something about typing in my prompts in a chatroom feels comfortingly intimate. All my questions suddenly have elaborate answers, without needing to endlessly comb through sources and webpages and having to think for myself.
When ChatGPT was first made publicly available in November 2022, I remember talking to friends about it, with the consensus arising that Large Language Models (LLM) seem to be exciting and fun. Still, nobody would have trusted it. Though the demographic data is still somewhat limited, we now see that in different countries worldwide, most students have a positive attitude towards using LLMs and report frequent usage (Castillo et al., 2023; Fazal et al., 2023). And not without good reason, generative AI takes charge of labour-intensive and tedious writing tasks; it handles ambiguous, unstructured input, filters through noise, summarizes, annotates, etc. (Yang et al., 2024). It does everything we usually would not be itching to do.
As LLMs become a mainstream tool in academia, their influence starts to become more noticeable in subtle but significant ways—especially in the language we use. The story that first made me aware of this phenomenon was the genius investigation of the “delve dilemma”. This large-scale analysis of scientific publications spanning 1849 to 2024 by Masukume (2024) revealed a noticeable spike in the use of certain words, such as “delve,” “realm,” and “underscore,” between 2023 and 2024. These terms co-occurred exclusively within this timeframe. Given their strong association with LLM-generated text, this finding serves as profound evidence of AI chatbots” growing impact on academic writing. LLMs favour certain vocabulary, humans adopt it, and over time, it becomes deeply embedded in our communication, ultimately reshaping the way we write and express ideas.
“Given their strong association with LLM-generated text, this finding serves as profound evidence of AI chatbots' growing impact on academic writing. LLMs favour certain vocabulary, humans adopt it, and over time, it becomes deeply embedded in our communication, ultimately reshaping the way we write and express ideas.”
This growing influence of LLMs on academic language reflects a broader challenge: the blurring lines between AI-generated and human-written text. Research has shown that people are often unable to distinguish between AI-generated and human-generated text, sometimes even leading to AI-generated text being perceived as “more human than human” (Jackesch et al., 2023). Jakesch et al., 2023 argue that this happens due to our often misguided conceptions about what AI-generated content should look like. For instance, personal statements like “I love spending weekends with my family” are assumed to be written by humans because they reference relatable life experiences, even though AI can easily generate such content. A warm tone or flawless grammar, such as “I am excited to create meaningful connections”, is often thought to be proof of human authorship, while errors or monotony are misattributed to either humans or poor AI. Finally, reflective phrases like “I have managed campaigns for five years” are mistaken for human writing due to their focus on the past, despite being easily replicable by AI systems.
“AI can replicate stylistic elements but, it will always remain tethered to its training data. Human fallibility is a cornerstone of the human experience, and even though AI itself is also faulty, rather than making novel mistakes, it primarily replicates existing misinformation.”
When we do recognize that text was AI-generated, we often fall victim to the automation bias – a tendency to trust AI-generated output blindly and uncritically. This mainly happens because we strongly believe that Large Language Models are objective agents who report without biases (Knapton, 2024). However, studies such as those by Van Niekerk et al. (2024) show how LLMs like GPT-2, ChatGPT, and Meta’s Llama 2 inherit and replicate certain gender and racial biases. For instance, during a hiring process, some models favour female candidates over equally qualified male candidates while assigning disproportionately lower scores to Black male candidates (An et al., 2024). Considering that they are largely trained on publicly available “human-made” texts and that humans exhibit biases, it makes sense for them to be biased as well. So, taking responses with a grain of salt is essential as they may not be as objective as we might intuitively think they are. Besides, relying on AI for answers ultimately puts us at the risk of stripping away a certain flawed humanness of communication and writing. Large Language Models offer polished but predictable outputs, lacking unfiltered creativity that can lead to truly original ideas or unconventional approaches to problems. AI can replicate stylistic elements but, it will always remain tethered to its training data. Human fallibility is a cornerstone of the human experience, and even though AI itself is also faulty, rather than making novel mistakes, it primarily replicates existing misinformation.
Today, there are substantial benefits to using ChatGPT, regardless of whether or not you choose to embrace them. At their core, they aim to provide efficient answers to our questions and are insightful tools. However, as we start to integrate AI into our everyday lives more and more, it is imperative that we remain vigilant about how it shapes us—our language, biases, and understanding of the world.
References
- An, J., Huang, D., Lin, C., & Tai, M. (2024). Measuring Gender and Racial Biases in Large Language Models.
- Castillo, A., Serna, G., S., Arocutipa, J., Berrios, H., Rodriguez, M., Reyes, G., Lopez, H., Teves, R., , H., Rivera, V., & Arias-Gonzáles, J. (2023). Effect of Chat GPT on the digitized learning process of university students. Journal of Namibian Studies : History Politics Culture. https://doi.org/10.59670/jns.v33i.411.
- Fazal, N., Qasmieh, S., & Graefen, B. (2023). Trick or Treat-Use of ChatGPT in Education.. The Journal of Immunology. https://doi.org/10.4049/jimmunol.210.supp.232.01.
- Jakesch, M., Hancock, J. T., & Naaman, M. (2023). Human heuristics for AI-generated language are flawed. Proceedings of the National Academy of Sciences, 120(11). https://doi.org/10.1073/pnas.2208839120
- Knapton, K. (2024, August 13). Navigating the Biases in LLM Generative AI: A Guide to Responsible Implementation. Forbes. https://www.forbes.com/councils/forbestechcouncil/2023/09/06/navigating-the-biases-in-llm-generative-ai-a-guide-to-responsible-implementation/
- Masukume, G. (2024). The Impact of AI on Scientific Literature: A surge in AI-Associated words in academic and biomedical writing. medRxiv (Cold Spring Harbor Laboratory). https://doi.org/10.1101/2024.05.31.24308296
- Romero-Rodríguez, J., Ramírez-Montoya, M., Buenestado-Fernández, M., & Lara-Lara, F. (2023). Use of ChatGPT at University as a Tool for Complex Thinking: Students” Perceived Usefulness. Journal of New Approaches in Educational Research. https://doi.org/10.7821/naer.2023.7.1458.
- Van Niekerk, D., et al. (2024). Challenging systematic prejudices: An investigation into gender bias in large language models. UNESCO and IRCAI. https://creativecommons.org/licenses/by-sa/3.0/igo/
- Yang, J., Jin, H., Tang, R., Han, X., Feng, Q., Jiang, H., Zhong, S., Yin, B., & Hu, X. (2024). Harnessing the power of LLMs in Practice: A survey on ChatGPT and Beyond. ACM Transactions on Knowledge Discovery From Data, 18(6), 1–32. https://doi.org/10.1145/3649506
No matter the task, assignment or question, ChatGPT has become my new Google. In some way, I cannot even equate it with Google – I would never converse with Google the same way – something about typing in my prompts in a chatroom feels comfortingly intimate. All my questions suddenly have elaborate answers, without needing to endlessly comb through sources and webpages and having to think for myself.
When ChatGPT was first made publicly available in November 2022, I remember talking to friends about it, with the consensus arising that Large Language Models (LLM) seem to be exciting and fun. Still, nobody would have trusted it. Though the demographic data is still somewhat limited, we now see that in different countries worldwide, most students have a positive attitude towards using LLMs and report frequent usage (Castillo et al., 2023; Fazal et al., 2023). And not without good reason, generative AI takes charge of labour-intensive and tedious writing tasks; it handles ambiguous, unstructured input, filters through noise, summarizes, annotates, etc. (Yang et al., 2024). It does everything we usually would not be itching to do.
As LLMs become a mainstream tool in academia, their influence starts to become more noticeable in subtle but significant ways—especially in the language we use. The story that first made me aware of this phenomenon was the genius investigation of the “delve dilemma”. This large-scale analysis of scientific publications spanning 1849 to 2024 by Masukume (2024) revealed a noticeable spike in the use of certain words, such as “delve,” “realm,” and “underscore,” between 2023 and 2024. These terms co-occurred exclusively within this timeframe. Given their strong association with LLM-generated text, this finding serves as profound evidence of AI chatbots” growing impact on academic writing. LLMs favour certain vocabulary, humans adopt it, and over time, it becomes deeply embedded in our communication, ultimately reshaping the way we write and express ideas.
“Given their strong association with LLM-generated text, this finding serves as profound evidence of AI chatbots' growing impact on academic writing. LLMs favour certain vocabulary, humans adopt it, and over time, it becomes deeply embedded in our communication, ultimately reshaping the way we write and express ideas.”
This growing influence of LLMs on academic language reflects a broader challenge: the blurring lines between AI-generated and human-written text. Research has shown that people are often unable to distinguish between AI-generated and human-generated text, sometimes even leading to AI-generated text being perceived as “more human than human” (Jackesch et al., 2023). Jakesch et al., 2023 argue that this happens due to our often misguided conceptions about what AI-generated content should look like. For instance, personal statements like “I love spending weekends with my family” are assumed to be written by humans because they reference relatable life experiences, even though AI can easily generate such content. A warm tone or flawless grammar, such as “I am excited to create meaningful connections”, is often thought to be proof of human authorship, while errors or monotony are misattributed to either humans or poor AI. Finally, reflective phrases like “I have managed campaigns for five years” are mistaken for human writing due to their focus on the past, despite being easily replicable by AI systems.
“AI can replicate stylistic elements but, it will always remain tethered to its training data. Human fallibility is a cornerstone of the human experience, and even though AI itself is also faulty, rather than making novel mistakes, it primarily replicates existing misinformation.”
When we do recognize that text was AI-generated, we often fall victim to the automation bias – a tendency to trust AI-generated output blindly and uncritically. This mainly happens because we strongly believe that Large Language Models are objective agents who report without biases (Knapton, 2024). However, studies such as those by Van Niekerk et al. (2024) show how LLMs like GPT-2, ChatGPT, and Meta’s Llama 2 inherit and replicate certain gender and racial biases. For instance, during a hiring process, some models favour female candidates over equally qualified male candidates while assigning disproportionately lower scores to Black male candidates (An et al., 2024). Considering that they are largely trained on publicly available “human-made” texts and that humans exhibit biases, it makes sense for them to be biased as well. So, taking responses with a grain of salt is essential as they may not be as objective as we might intuitively think they are. Besides, relying on AI for answers ultimately puts us at the risk of stripping away a certain flawed humanness of communication and writing. Large Language Models offer polished but predictable outputs, lacking unfiltered creativity that can lead to truly original ideas or unconventional approaches to problems. AI can replicate stylistic elements but, it will always remain tethered to its training data. Human fallibility is a cornerstone of the human experience, and even though AI itself is also faulty, rather than making novel mistakes, it primarily replicates existing misinformation.
Today, there are substantial benefits to using ChatGPT, regardless of whether or not you choose to embrace them. At their core, they aim to provide efficient answers to our questions and are insightful tools. However, as we start to integrate AI into our everyday lives more and more, it is imperative that we remain vigilant about how it shapes us—our language, biases, and understanding of the world.
References
- An, J., Huang, D., Lin, C., & Tai, M. (2024). Measuring Gender and Racial Biases in Large Language Models.
- Castillo, A., Serna, G., S., Arocutipa, J., Berrios, H., Rodriguez, M., Reyes, G., Lopez, H., Teves, R., , H., Rivera, V., & Arias-Gonzáles, J. (2023). Effect of Chat GPT on the digitized learning process of university students. Journal of Namibian Studies : History Politics Culture. https://doi.org/10.59670/jns.v33i.411.
- Fazal, N., Qasmieh, S., & Graefen, B. (2023). Trick or Treat-Use of ChatGPT in Education.. The Journal of Immunology. https://doi.org/10.4049/jimmunol.210.supp.232.01.
- Jakesch, M., Hancock, J. T., & Naaman, M. (2023). Human heuristics for AI-generated language are flawed. Proceedings of the National Academy of Sciences, 120(11). https://doi.org/10.1073/pnas.2208839120
- Knapton, K. (2024, August 13). Navigating the Biases in LLM Generative AI: A Guide to Responsible Implementation. Forbes. https://www.forbes.com/councils/forbestechcouncil/2023/09/06/navigating-the-biases-in-llm-generative-ai-a-guide-to-responsible-implementation/
- Masukume, G. (2024). The Impact of AI on Scientific Literature: A surge in AI-Associated words in academic and biomedical writing. medRxiv (Cold Spring Harbor Laboratory). https://doi.org/10.1101/2024.05.31.24308296
- Romero-Rodríguez, J., Ramírez-Montoya, M., Buenestado-Fernández, M., & Lara-Lara, F. (2023). Use of ChatGPT at University as a Tool for Complex Thinking: Students” Perceived Usefulness. Journal of New Approaches in Educational Research. https://doi.org/10.7821/naer.2023.7.1458.
- Van Niekerk, D., et al. (2024). Challenging systematic prejudices: An investigation into gender bias in large language models. UNESCO and IRCAI. https://creativecommons.org/licenses/by-sa/3.0/igo/
- Yang, J., Jin, H., Tang, R., Han, X., Feng, Q., Jiang, H., Zhong, S., Yin, B., & Hu, X. (2024). Harnessing the power of LLMs in Practice: A survey on ChatGPT and Beyond. ACM Transactions on Knowledge Discovery From Data, 18(6), 1–32. https://doi.org/10.1145/3649506