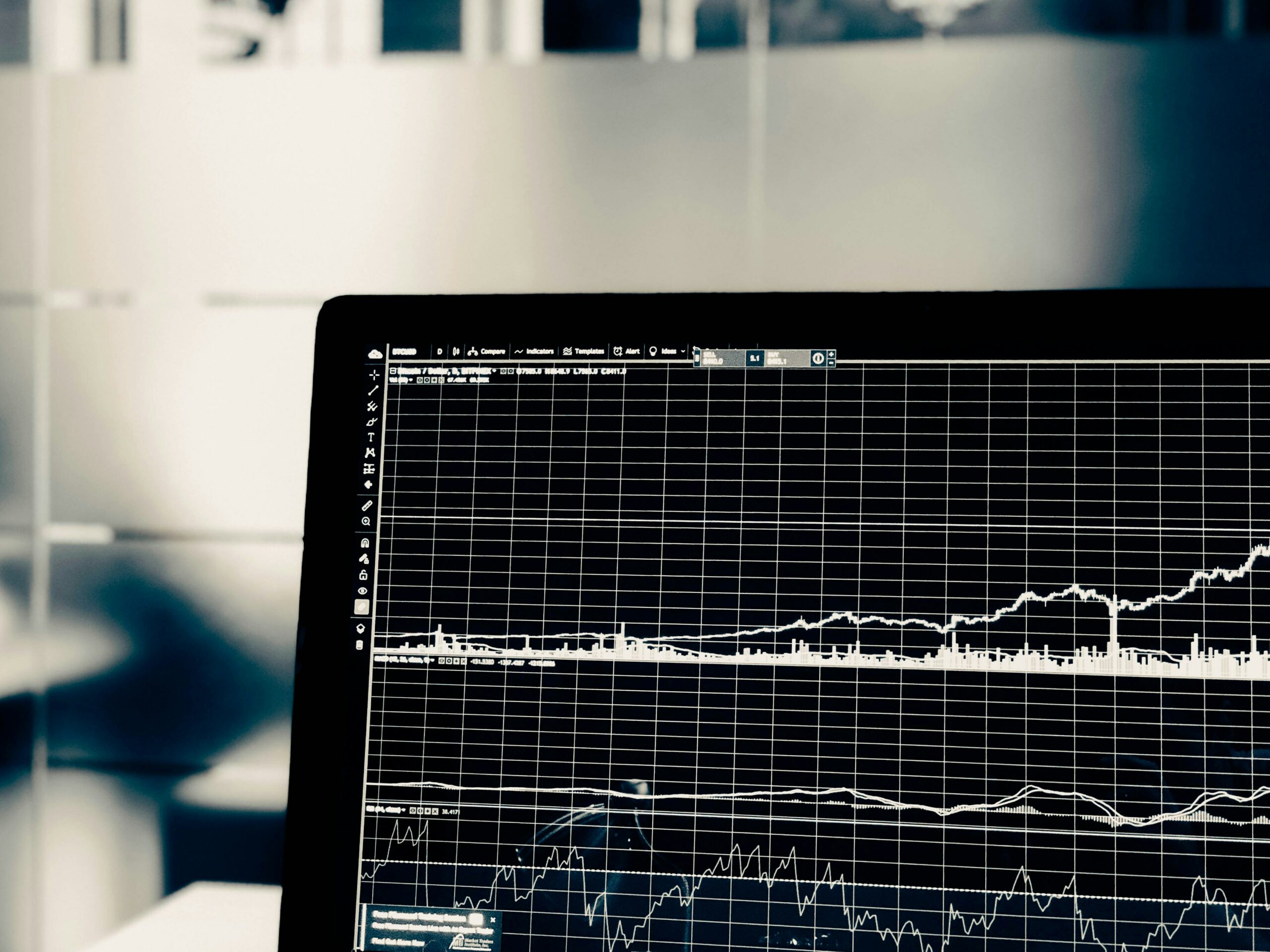
With recent artificial intelligence (AI) developments dominating headlines and discussions, people and organizations are rushing to integrate it into various aspects of life. AI systems have made the leap from performing routine tasks (e.g., customer service chatbots, forecasting) to supervising human employees (Technica, 2024; Lanz et al., 2024). For example, gig economy companies such as Uber commonly rely on AI to instruct their workers, and even punish them for alleged misbehavior (e.g., automated bans for low driver rating, or low passenger acceptance rates) (Möhlmann & Zalmanson, 2017; Möhlmann et al., 2021; Lanz et al., 2024).
With recent artificial intelligence (AI) developments dominating headlines and discussions, people and organizations are rushing to integrate it into various aspects of life. AI systems have made the leap from performing routine tasks (e.g., customer service chatbots, forecasting) to supervising human employees (Technica, 2024; Lanz et al., 2024). For example, gig economy companies such as Uber commonly rely on AI to instruct their workers, and even punish them for alleged misbehavior (e.g., automated bans for low driver rating, or low passenger acceptance rates) (Möhlmann & Zalmanson, 2017; Möhlmann et al., 2021; Lanz et al., 2024).
Photo by Chris Liverani
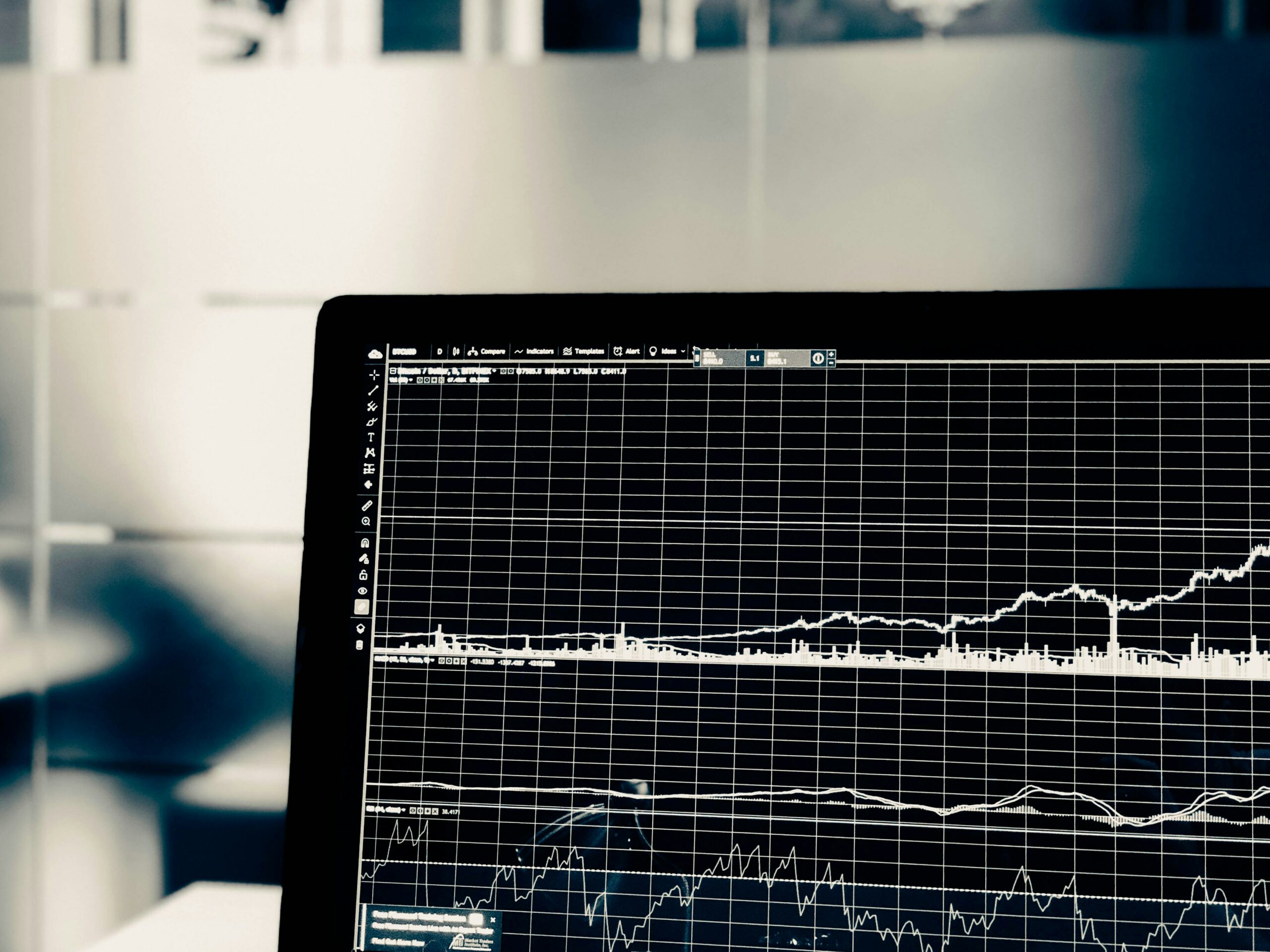
Photo by Chris Liverani
Automation Bias
A possible psychological mechanism that can explain the corporate hype around automated technologies and AI is automation bias. Automation bias occurs when users place too much trust in the reliability and accuracy of AI performance, often preferring algorithmic recommendations over human advice (i.e., algorithmic appreciation), leading to excessive dependence on the technology (Skitka, 2011; Jones-Jang & Park, 2023). However, while AI promises efficiency and innovation with its advanced capabilities, it is still a probabilistic model, working with estimates and sometimes making mistakes (Pascual, 2024). Rushing the implementation of AI in high-responsibility roles can lead to significant consequences, that are not merely hypothetical future concerns, but already present problems.
Cases of Misuse of AI systems
To name a few problems already created by irresponsible AI implementation, one can take a look at several media reports which revealed that prominent organizations had adopted AI applications that adversely affected marginalized groups. For example, Amazon was forced to scrap its AI recruiting tool after discovering it was biased against women, favoring applicants using “masculine language” in their resumes (Dastin, 2018; Lanz et al., 2024).
In public administration and policing, AI is advertised as able to provide efficient solutions and decrease workload. However, unchecked AI implementation has led to high-profile errors, such as the “Toeslagenaffaire” or the child care benefits scandal in the Netherlands (Amnesty International, 2021; Heikkilä, 2022). In 2013, Dutch tax authorities used a self-learning algorithm to create risk profiles for identifying child care benefits fraud. Indicators such as dual nationality or low income were incorrectly classified as risk factors by the algorithm. This resulted in harsh penalties: tens of thousands of often lower-income, ethnic-minority families being falsely accused of tax fraud and driven into poverty, leading to unemployment, debt, and forced evictions. Tragically, some victims committed suicide, and more than a thousand children were placed into foster care (Amnesty International, 2021; Heikkilä, 2022). Similarly, Amsterdam’s Top 400 and 600 programs, automated lists of people at risk of criminal behavior, are criticized for ethnic profiling and reinforcing institutional racism. A senior policy advisor at the European Digital Rights network (EDRi) says these automated risk modeling and profiling systems “treat everyone as suspects to some extent”, but “disproportionately targets racialized individuals, those perceived as migrants or terrorists, and people from poorer, working-class communities.” (Reuters, 2021).
“AI’s potential for greater efficiency is marred by the danger of accelerating the speed of violence when humans are reduced to data points.”
Perhaps most concerning is AI’s growing use in the military. Wopke Hoekstra, then-Minister of Foreign Affairs in the Netherlands, states: “The rise of AI is one of the greatest future challenges in international security and arms control.” (Informatie Rijksoverheid, 2023). During the Israeli military’s campaign in Gaza, reliance on their Gospel and Lavender AI systems, designed to track and bomb individuals marked as targets when they are home, are criticized for making killing more objective, resulting in, as of December 2023, more Palestinians killed than all previous Palestinian-Israeli clashes since the start of the First Intifada combined (Abraham, 2024; Byman et al., 2024; Elidrissi, 2024; Pascual, 2024; Pratt, 2024). AI’s potential for greater efficiency is marred by the danger of accelerating the speed of violence when humans are reduced to data points.
There is a fear that, since AI supervisors are designed to enhance performance, they might push employees towards unethical behavior if it appears beneficial for meeting the supervisor’s predefined objectives (Köbis et al., 2021). Although previous research indicates that current algorithms do not intentionally discriminate, but instead reproduce biases extracted from data they were trained on (Obermeyer et al., 2019; Lanz et al., 2024), the use of AI instead of a human supervisor may enable unethical actions by diffusing responsibility. In-line with automation bias, decision-makers might feel less responsible for the outcomes dictated by an algorithm perceived as more objective or efficient, thereby reducing their moral engagement (Köbis et al, 2021). Returning to the example of Amazon’s recruiting tool discriminating against women in hiring, retention, and promotion decisions, human HR employees often complied with these directives (Dastin, 2018; Lanz et al., 2024). In the Toeslagenaffaire, Dutch tax authorities were quick to penalize families for a period of 6 years over a mere suspicion of fraud based on the system’s risk indicators (Amnesty International, 2021; Heikkilä, 2022).
Human in the Loop: An inadequate solution
A popular solution against suboptimal AI implementation is the “human-in-the-loop” approach. The idea is human oversight can correct or mitigate errors in automated decision-making. However, this solution falls short when AI systems are designed to address manpower shortages or reduce efficiency bottlenecks. In such cases, the very constraints that necessitate AI implementation—such as limited time, personnel, and resources—make it impractical to rely on extensive human review for every decision (Franks, 2022; Streitfeld, 2024). For example, the Sensing Project, which aimed to counter crimes like shoplifting in the southeastern Dutch city of Roermond, was forced to end because the police did not have enough capacity to follow up project data (Reuters, 2021). An investigation by Jerusalem-based journalists in +972 Magazine and Local Call revealed, after the October 7th 2023 terrorist attacks, the Israeli military faced immense pressure to generate and act upon a high volume of targets in Gaza. The Lavender system was given a large margin of error, and human personnel were instructed to authorize bombings with minimal review, sometimes spending only around 20 seconds to verify the target was male, despite knowing that Lavender made errors in about 10 percent of cases. This was reported as one of the reasons why so many individuals, including Palestinian civilians who were not involved in militant activities, were targeted (Abraham, 2024; Elidrissi, 2024; Pascual, 2024; Pratt, 2024). Of course, while a human-in-the-loop can offer an additional layer of oversight, it alone cannot resolve the deeper institutional and systemic biases that may affect the human itself.
“In entrusting social and organizational databases to AI systems, we need to remember that every datapoint is a family too.”
Algorithmic aversion: A respite due to novelty?
Thankfully, people are often less forgiving of AI failures. Previous studies show that, due to a psychological mechanism called algorithmic aversion, participants react more strongly to AI inconsistencies, as these failures violate the perceived perfection created by automation bias. When AI systems demonstrate decreasing reliability, trust in them diminishes significantly faster than trust in human advisers (Glikson & Woolley, 2020; Jones-Jang & Park, 2023). Studies suggest these trends to be caused by the novelty of current AI technologies (Ulfert-Blank et al., 2023). American cities, such as Santa Cruz and New Orleans, are already banning AI policing systems amid accusations that they reinforce racist policing patterns, and the European Union is positioned to take the lead towards implementing a risk-based model for regulating AI (Reuters, 2021; 2024). As future generations grow up with AI, they may become less cautious of its limitations. The lack of diversity in current AI regulatory board compositions (eg. Meta’s AI advisory council is composed entirely of White men), reflects existing societal biases which are set to be perpetuated in AI governance, further entrenching systemic biases in the future (Vlasceanu & Amodio, 2022; Duffy, 2024).
As countries race to increase investment in AI as a competitive advantage in the civil and military sector (Fiesler, 2023; Informatie Rijksoverheid, 2023), governments should also invest in crucial academics and watchdog or research organizations such as Bits of Freedom, an independent Dutch digital rights foundation, focusing on privacy and communications freedom, the Distributed AI Research (DAIR), founded by the ex-co-leader of Google’s Ethical A.I. team, and the Intimacies of Remote Warfare (IRW) programme by Utrecht University (Bits of Freedom, 2016; Walsh, 2022; Intimacies of Remote Warfare | Universiteit Utrecht, 2024). These organizations should be supported in their crucial role of educating the public and government sectors about overzealous AI development.
While AI promises to transform various sectors, the rush to deploy AI in high-stakes roles can lead to dehumanizing outcomes. Responsible AI development involves not only checking for biases and accuracy, but also understanding appropriate and beneficial applications for the humans interacting with these systems (Fiesler, 2024). In entrusting social and organizational databases to AI systems, we need to remember that every datapoint is a family too. Casey Fiesler, an Associate Professor of Information Science at University of Colorado Boulder, highlights a quote from The Conversation: “Automation bias cedes moral authority to the dispassionate interface of statistical processing.” (Schwarz, 2024). We must remain vigilant to prevent automation bias from eroding our moral responsibility and authority when we enter a future increasingly dominated by AI.
References
- Abraham, Y. (2024, April 3). ‘Lavender’: The AI machine directing Israel’s bombing spree in Gaza. +972 Magazine. https://www.972mag.com/lavender-ai-israeli-army-gaza/
- Amnesty International. (2021, November 1). Dutch childcare benefit scandal an urgent wake-up call to ban racist algorithms. https://www.amnesty.org/en/latest/news/2021/10/xenophobic-machines-dutch-child-benefit-scandal/
- Bits of Freedom. (2016, January 27). Member Spotlight: Bits of Freedom – European Digital Rights (EDRI). European Digital Rights (EDRi). https://edri.org/our-work/member-spotlight-bits-of-freedom/
- Byman, D., McCabe, R., Palmer, A., Doxsee, C., Holtz, M., & Duff, D. (2024, January 5). Hamas’s October 7 attack: Visualizing the data. csis.org. https://www.csis.org/analysis/hamass-october-7-attack-visualizing-data
- Dastin, J. (2018, Oct 11). Amazon scraps secret AI recruiting tool that showed bias against women. Reuters Media. Retrieved from https://www.reuters.com/article/us-amazon-com-jobs-autom ation-insight-idUSKCN1MK08G
- Duffy, C. (2024, May 23). Meta created an AI advisory council that’s composed entirely of white men. CNN. https://edition.cnn.com/2024/05/23/tech/meta-ai-adivsory-council-white-men/index.html
- Elidrissi, R. (2024, May 7). How AI tells Israel who to bomb. Vox. https://www.vox.com/videos/24151531/israel-ai-gaza-gospel-lavender
- Fiesler, C. (2023, June 22). AI has social consequences, but who pays the price? Tech companies’ problem with ‘ethical debt.’ Medium. https://medium.com/the-conversation/ai-has-social-consequences-but-who-pays-the-price-tech-companies-problem-with-ethical-debt-d685bab5859f
- Fiesler, C. (2024, May 20). Casey Fiesler on LinkedIn: AI chatbots are intruding into online communities where people are trying. . . | 26 comments. https://www.linkedin.com/posts/casey-fiesler-bb3493243_ai-chatbots-are-intruding-into-online-communities-activity-7198322637779947520-2wfX
- Franks, B. (2022a, January 5). Is human in the loop AI doomed? – Analytics Matters – medium. Medium. https://medium.com/analytics-matters/human-in-the-loop-is-a-popular-way-to-mitigate-the-risks-of-ai-that-approach-might-be-doomed-f6631cd1e78d
- Glikson, E., & Woolley, A. W. (2020). Human Trust in Artificial Intelligence: Review of Empirical Research. Academy of Management Annals, 14(2), 627–660. https://doi.org/10.5465/annals.2018.0057
- Heikkilä, M. (2022, April 13). Dutch scandal serves as a warning for Europe over risks of using algorithms. POLITICO. https://www.politico.eu/article/dutch-scandal-serves-as-a-warning-for-europe-over-risks-of-using-algorithms/#:~:text=In%202019%20it%20was%20revealed,on%20the%20system’s%20risk%20indicators
- Informatie Rijksoverheid. (2023, February 21). About REAIM 2023. Ministry of Foreign Affairs | Government.nl. https://www.government.nl/ministries/ministry-of-foreign-affairs/activiteiten/reaim/about-reaim-2023#:~:text=REAIM%202023%20took%20place%20at,vulnerabilities%20associated%20with%20military%20AI
- Intimacies of Remote Warfare | Universiteit Utrecht. (2024, July 15). Intimacies of Remote Warfare – Universiteit Utrecht. https://intimacies-of-remote-warfare.nl/
- Instagram. (n.d.). https://www.instagram.com/reel/C6mLlKOLTwe/?utm_source=ig_web_copy_link
- Jones-Jang, S. M., & Park, Y. J. (2023). How do people react to AI failure? Automation bias, algorithmic aversion, and perceived controllability. Journal of Computer-Mediated Communication, 28(1), zmac029. https://doi.org/10.1093/jcmc/zmac029
- Köbis, N., Bonnefon, J.-F., & Rahwan, I. (2021). Bad machines corrupt good morals. Nature Human Behaviour, 5(6), 679–685. https:// doi.org/10.1038/s41562-021-01128-2
- Military is the missing word in AI safety discussions. (n.d.). Financial Times. https://www.ft.com/content/da03f8e1-0ae4-452d-acd1-ec284b6acd78
- Ministerie van Algemene Zaken. (2023, February 21). About REAIM 2023. Ministry of Foreign Affairs | Government.nl. https://www.government.nl/ministries/ministry-of-foreign-affairs/activiteiten/reaim/about-reaim-2023#:~:text=REAIM%202023%20took%20place%20at,vulnerabilities%20associated%20with%20military%20AI.
- Möhlmann, M., & Zalmanson, L. (2017, December). Hands on the wheel: Navigating algorithmic management and Uber drivers’. In Autonomy’, in proceedings of the international conference on information systems (ICIS), Seoul South Korea (pp. 10-13). https://aisel.aisnet.org/icis2017/DigitalPlatforms/Presentations/3
- Möhlmann, M., Zalmanson, L., Henfridsson, O., & Gregory, R. W. (2021). Algorithmic management of work on online labor platforms: When matching meets control. MIS quarterly, 45(4). https://doi.org/10.25300/MISQ/2021/15333
- Obermeyer, Z., Powers, B., Vogeli, C., & Mullainathan, S. (2019). Dissecting racial bias in an algorithm used to manage the health of populations. Science, 366(6464), 447–453. https://doi.org/10. 1126/science.aax2342
- Lanz, L., Briker, R., & Gerpott, F. H. (2024). Employees adhere more to unethical instructions from human than AI supervisors: Complementing experimental evidence with machine learning. Journal of Business Ethics, 189(3), 625-646. https://doi.org/10.1007/s10551-023-05393-1
- Pascual, M. G. (2024, April 17). Lavender, Israel’s artificial intelligence system that decides who to bomb in Gaza. EL PAÍS English. https://english.elpais.com/technology/2024-04-17/lavender-israels-artificial-intelligence-system-that-decides-who-to-bomb-in-gaza.html
- Pratt, S. F. (2024, May 4). Israel’s algorithmic killing of Palestinians sets dangerous precedent. Foreign Policy. https://foreignpolicy.com/2024/05/02/israel-military-artificial-intelligence-targeting-hamas-gaza-deaths-lavender/
- Reuters. (2021, October 25). Controversial technology. Copyright 2021 by the Global Times. https://www.globaltimes.cn/page/202110/1237232.shtml
- Reuters. (2024, January 18). Dutch to use Europe’s AI Act “immediately,” invest $222 mln in sector. Reuters. https://www.reuters.com/technology/dutch-use-europes-ai-act-immediately-invest-222-mln-sector-2024-01-18/
- Schwarz, E. (2024, April 12). Gaza war: Israel using AI to identify human targets raising fears that innocents are being caught in the net. The Conversation. https://theconversation.com/gaza-war-israel-using-ai-to-identify-human-targets-raising-fears-that-innocents-are-being-caught-in-the-net-227422
- Skitka, L. (2011). Automation Bias | Linda J. Skitka, Ph.D. https://lskitka.people.uic.edu/. Retrieved September 1, 2024, from https://lskitka.people.uic.edu/styled-7/styled-14/#:~:text=Automation%20bias%20refers%20to%20a,to%20monitor%20on%2Dgoing%20tasks.
- Streitfeld, D. (2024, May 30). If AI can do your job, maybe it can also replace your CEO. The Business Times. https://www.businesstimes.com.sg/working-life/if-ai-can-do-your-job-maybe-it-can-also-replace-your-ceo
- Technica, A. B. A. (2024, February 17). Air Canada has to honor a refund policy its chatbot made up. WIRED. https://www.wired.com/story/air-canada-chatbot-refund-policy/
- Ulfert-Blank, A. S., Georganta, E., Tielman, M., & Oron-Gilad, T. (2023, January). Piecing Together the Puzzle: Understanding Trust in Human-AI Teams. In CEUR Workshop Proceedings (Vol. 3456, pp. 169-174). CEUR-WS. https://ceur-ws.org/Vol-3456/
- Vlasceanu, M., & Amodio, D. M. (2022). Propagation of societal gender inequality by internet search algorithms. Proceedings of the National Academy of Sciences, 119(29),e2204529119. https://doi.org/10.1073/pnas.2204529119
- Walsh, D. (2022, May 26). Timnit Gebru: Ethical AI requires institutional and structural change. Stanford HAI. https://hai.stanford.edu/news/timnit-gebru-ethical-ai-requires-institutional-and-structural-change
Automation Bias
A possible psychological mechanism that can explain the corporate hype around automated technologies and AI is automation bias. Automation bias occurs when users place too much trust in the reliability and accuracy of AI performance, often preferring algorithmic recommendations over human advice (i.e., algorithmic appreciation), leading to excessive dependence on the technology (Skitka, 2011; Jones-Jang & Park, 2023). However, while AI promises efficiency and innovation with its advanced capabilities, it is still a probabilistic model, working with estimates and sometimes making mistakes (Pascual, 2024). Rushing the implementation of AI in high-responsibility roles can lead to significant consequences, that are not merely hypothetical future concerns, but already present problems.
Cases of Misuse of AI systems
To name a few problems already created by irresponsible AI implementation, one can take a look at several media reports which revealed that prominent organizations had adopted AI applications that adversely affected marginalized groups. For example, Amazon was forced to scrap its AI recruiting tool after discovering it was biased against women, favoring applicants using “masculine language” in their resumes (Dastin, 2018; Lanz et al., 2024).
In public administration and policing, AI is advertised as able to provide efficient solutions and decrease workload. However, unchecked AI implementation has led to high-profile errors, such as the “Toeslagenaffaire” or the child care benefits scandal in the Netherlands (Amnesty International, 2021; Heikkilä, 2022). In 2013, Dutch tax authorities used a self-learning algorithm to create risk profiles for identifying child care benefits fraud. Indicators such as dual nationality or low income were incorrectly classified as risk factors by the algorithm. This resulted in harsh penalties: tens of thousands of often lower-income, ethnic-minority families being falsely accused of tax fraud and driven into poverty, leading to unemployment, debt, and forced evictions. Tragically, some victims committed suicide, and more than a thousand children were placed into foster care (Amnesty International, 2021; Heikkilä, 2022). Similarly, Amsterdam’s Top 400 and 600 programs, automated lists of people at risk of criminal behavior, are criticized for ethnic profiling and reinforcing institutional racism. A senior policy advisor at the European Digital Rights network (EDRi) says these automated risk modeling and profiling systems “treat everyone as suspects to some extent”, but “disproportionately targets racialized individuals, those perceived as migrants or terrorists, and people from poorer, working-class communities.” (Reuters, 2021).
“AI’s potential for greater efficiency is marred by the danger of accelerating the speed of violence when humans are reduced to data points.”
Perhaps most concerning is AI’s growing use in the military. Wopke Hoekstra, then-Minister of Foreign Affairs in the Netherlands, states: “The rise of AI is one of the greatest future challenges in international security and arms control.” (Informatie Rijksoverheid, 2023). During the Israeli military’s campaign in Gaza, reliance on their Gospel and Lavender AI systems, designed to track and bomb individuals marked as targets when they are home, are criticized for making killing more objective, resulting in, as of December 2023, more Palestinians killed than all previous Palestinian-Israeli clashes since the start of the First Intifada combined (Abraham, 2024; Byman et al., 2024; Elidrissi, 2024; Pascual, 2024; Pratt, 2024). AI’s potential for greater efficiency is marred by the danger of accelerating the speed of violence when humans are reduced to data points.
There is a fear that, since AI supervisors are designed to enhance performance, they might push employees towards unethical behavior if it appears beneficial for meeting the supervisor’s predefined objectives (Köbis et al., 2021). Although previous research indicates that current algorithms do not intentionally discriminate, but instead reproduce biases extracted from data they were trained on (Obermeyer et al., 2019; Lanz et al., 2024), the use of AI instead of a human supervisor may enable unethical actions by diffusing responsibility. In-line with automation bias, decision-makers might feel less responsible for the outcomes dictated by an algorithm perceived as more objective or efficient, thereby reducing their moral engagement (Köbis et al, 2021). Returning to the example of Amazon’s recruiting tool discriminating against women in hiring, retention, and promotion decisions, human HR employees often complied with these directives (Dastin, 2018; Lanz et al., 2024). In the Toeslagenaffaire, Dutch tax authorities were quick to penalize families for a period of 6 years over a mere suspicion of fraud based on the system’s risk indicators (Amnesty International, 2021; Heikkilä, 2022).
Human in the Loop: An inadequate solution
A popular solution against suboptimal AI implementation is the “human-in-the-loop” approach. The idea is human oversight can correct or mitigate errors in automated decision-making. However, this solution falls short when AI systems are designed to address manpower shortages or reduce efficiency bottlenecks. In such cases, the very constraints that necessitate AI implementation—such as limited time, personnel, and resources—make it impractical to rely on extensive human review for every decision (Franks, 2022; Streitfeld, 2024). For example, the Sensing Project, which aimed to counter crimes like shoplifting in the southeastern Dutch city of Roermond, was forced to end because the police did not have enough capacity to follow up project data (Reuters, 2021). An investigation by Jerusalem-based journalists in +972 Magazine and Local Call revealed, after the October 7th 2023 terrorist attacks, the Israeli military faced immense pressure to generate and act upon a high volume of targets in Gaza. The Lavender system was given a large margin of error, and human personnel were instructed to authorize bombings with minimal review, sometimes spending only around 20 seconds to verify the target was male, despite knowing that Lavender made errors in about 10 percent of cases. This was reported as one of the reasons why so many individuals, including Palestinian civilians who were not involved in militant activities, were targeted (Abraham, 2024; Elidrissi, 2024; Pascual, 2024; Pratt, 2024). Of course, while a human-in-the-loop can offer an additional layer of oversight, it alone cannot resolve the deeper institutional and systemic biases that may affect the human itself.
“In entrusting social and organizational databases to AI systems, we need to remember that every datapoint is a family too.”
Algorithmic aversion: A respite due to novelty?
Thankfully, people are often less forgiving of AI failures. Previous studies show that, due to a psychological mechanism called algorithmic aversion, participants react more strongly to AI inconsistencies, as these failures violate the perceived perfection created by automation bias. When AI systems demonstrate decreasing reliability, trust in them diminishes significantly faster than trust in human advisers (Glikson & Woolley, 2020; Jones-Jang & Park, 2023). Studies suggest these trends to be caused by the novelty of current AI technologies (Ulfert-Blank et al., 2023). American cities, such as Santa Cruz and New Orleans, are already banning AI policing systems amid accusations that they reinforce racist policing patterns, and the European Union is positioned to take the lead towards implementing a risk-based model for regulating AI (Reuters, 2021; 2024). As future generations grow up with AI, they may become less cautious of its limitations. The lack of diversity in current AI regulatory board compositions (eg. Meta’s AI advisory council is composed entirely of White men), reflects existing societal biases which are set to be perpetuated in AI governance, further entrenching systemic biases in the future (Vlasceanu & Amodio, 2022; Duffy, 2024).
As countries race to increase investment in AI as a competitive advantage in the civil and military sector (Fiesler, 2023; Informatie Rijksoverheid, 2023), governments should also invest in crucial academics and watchdog or research organizations such as Bits of Freedom, an independent Dutch digital rights foundation, focusing on privacy and communications freedom, the Distributed AI Research (DAIR), founded by the ex-co-leader of Google’s Ethical A.I. team, and the Intimacies of Remote Warfare (IRW) programme by Utrecht University (Bits of Freedom, 2016; Walsh, 2022; Intimacies of Remote Warfare | Universiteit Utrecht, 2024). These organizations should be supported in their crucial role of educating the public and government sectors about overzealous AI development.
While AI promises to transform various sectors, the rush to deploy AI in high-stakes roles can lead to dehumanizing outcomes. Responsible AI development involves not only checking for biases and accuracy, but also understanding appropriate and beneficial applications for the humans interacting with these systems (Fiesler, 2024). In entrusting social and organizational databases to AI systems, we need to remember that every datapoint is a family too. Casey Fiesler, an Associate Professor of Information Science at University of Colorado Boulder, highlights a quote from The Conversation: “Automation bias cedes moral authority to the dispassionate interface of statistical processing.” (Schwarz, 2024). We must remain vigilant to prevent automation bias from eroding our moral responsibility and authority when we enter a future increasingly dominated by AI. <<
References
- Abraham, Y. (2024, April 3). ‘Lavender’: The AI machine directing Israel’s bombing spree in Gaza. +972 Magazine. https://www.972mag.com/lavender-ai-israeli-army-gaza/
- Amnesty International. (2021, November 1). Dutch childcare benefit scandal an urgent wake-up call to ban racist algorithms. https://www.amnesty.org/en/latest/news/2021/10/xenophobic-machines-dutch-child-benefit-scandal/
- Bits of Freedom. (2016, January 27). Member Spotlight: Bits of Freedom – European Digital Rights (EDRI). European Digital Rights (EDRi). https://edri.org/our-work/member-spotlight-bits-of-freedom/
- Byman, D., McCabe, R., Palmer, A., Doxsee, C., Holtz, M., & Duff, D. (2024, January 5). Hamas’s October 7 attack: Visualizing the data. csis.org. https://www.csis.org/analysis/hamass-october-7-attack-visualizing-data
- Dastin, J. (2018, Oct 11). Amazon scraps secret AI recruiting tool that showed bias against women. Reuters Media. Retrieved from https://www.reuters.com/article/us-amazon-com-jobs-autom ation-insight-idUSKCN1MK08G
- Duffy, C. (2024, May 23). Meta created an AI advisory council that’s composed entirely of white men. CNN. https://edition.cnn.com/2024/05/23/tech/meta-ai-adivsory-council-white-men/index.html
- Elidrissi, R. (2024, May 7). How AI tells Israel who to bomb. Vox. https://www.vox.com/videos/24151531/israel-ai-gaza-gospel-lavender
- Fiesler, C. (2023, June 22). AI has social consequences, but who pays the price? Tech companies’ problem with ‘ethical debt.’ Medium. https://medium.com/the-conversation/ai-has-social-consequences-but-who-pays-the-price-tech-companies-problem-with-ethical-debt-d685bab5859f
- Fiesler, C. (2024, May 20). Casey Fiesler on LinkedIn: AI chatbots are intruding into online communities where people are trying. . . | 26 comments. https://www.linkedin.com/posts/casey-fiesler-bb3493243_ai-chatbots-are-intruding-into-online-communities-activity-7198322637779947520-2wfX
- Franks, B. (2022a, January 5). Is human in the loop AI doomed? – Analytics Matters – medium. Medium. https://medium.com/analytics-matters/human-in-the-loop-is-a-popular-way-to-mitigate-the-risks-of-ai-that-approach-might-be-doomed-f6631cd1e78d
- Glikson, E., & Woolley, A. W. (2020). Human Trust in Artificial Intelligence: Review of Empirical Research. Academy of Management Annals, 14(2), 627–660. https://doi.org/10.5465/annals.2018.0057
- Heikkilä, M. (2022, April 13). Dutch scandal serves as a warning for Europe over risks of using algorithms. POLITICO. https://www.politico.eu/article/dutch-scandal-serves-as-a-warning-for-europe-over-risks-of-using-algorithms/#:~:text=In%202019%20it%20was%20revealed,on%20the%20system’s%20risk%20indicators
- Informatie Rijksoverheid. (2023, February 21). About REAIM 2023. Ministry of Foreign Affairs | Government.nl. https://www.government.nl/ministries/ministry-of-foreign-affairs/activiteiten/reaim/about-reaim-2023#:~:text=REAIM%202023%20took%20place%20at,vulnerabilities%20associated%20with%20military%20AI
- Intimacies of Remote Warfare | Universiteit Utrecht. (2024, July 15). Intimacies of Remote Warfare – Universiteit Utrecht. https://intimacies-of-remote-warfare.nl/
- Instagram. (n.d.). https://www.instagram.com/reel/C6mLlKOLTwe/?utm_source=ig_web_copy_link
- Jones-Jang, S. M., & Park, Y. J. (2023). How do people react to AI failure? Automation bias, algorithmic aversion, and perceived controllability. Journal of Computer-Mediated Communication, 28(1), zmac029. https://doi.org/10.1093/jcmc/zmac029
- Köbis, N., Bonnefon, J.-F., & Rahwan, I. (2021). Bad machines corrupt good morals. Nature Human Behaviour, 5(6), 679–685. https:// doi.org/10.1038/s41562-021-01128-2
- Military is the missing word in AI safety discussions. (n.d.). Financial Times. https://www.ft.com/content/da03f8e1-0ae4-452d-acd1-ec284b6acd78
- Ministerie van Algemene Zaken. (2023, February 21). About REAIM 2023. Ministry of Foreign Affairs | Government.nl. https://www.government.nl/ministries/ministry-of-foreign-affairs/activiteiten/reaim/about-reaim-2023#:~:text=REAIM%202023%20took%20place%20at,vulnerabilities%20associated%20with%20military%20AI.
- Möhlmann, M., & Zalmanson, L. (2017, December). Hands on the wheel: Navigating algorithmic management and Uber drivers’. In Autonomy’, in proceedings of the international conference on information systems (ICIS), Seoul South Korea (pp. 10-13). https://aisel.aisnet.org/icis2017/DigitalPlatforms/Presentations/3
- Möhlmann, M., Zalmanson, L., Henfridsson, O., & Gregory, R. W. (2021). Algorithmic management of work on online labor platforms: When matching meets control. MIS quarterly, 45(4). https://doi.org/10.25300/MISQ/2021/15333
- Obermeyer, Z., Powers, B., Vogeli, C., & Mullainathan, S. (2019). Dissecting racial bias in an algorithm used to manage the health of populations. Science, 366(6464), 447–453. https://doi.org/10. 1126/science.aax2342
- Lanz, L., Briker, R., & Gerpott, F. H. (2024). Employees adhere more to unethical instructions from human than AI supervisors: Complementing experimental evidence with machine learning. Journal of Business Ethics, 189(3), 625-646. https://doi.org/10.1007/s10551-023-05393-1
- Pascual, M. G. (2024, April 17). Lavender, Israel’s artificial intelligence system that decides who to bomb in Gaza. EL PAÍS English. https://english.elpais.com/technology/2024-04-17/lavender-israels-artificial-intelligence-system-that-decides-who-to-bomb-in-gaza.html
- Pratt, S. F. (2024, May 4). Israel’s algorithmic killing of Palestinians sets dangerous precedent. Foreign Policy. https://foreignpolicy.com/2024/05/02/israel-military-artificial-intelligence-targeting-hamas-gaza-deaths-lavender/
- Reuters. (2021, October 25). Controversial technology. Copyright 2021 by the Global Times. https://www.globaltimes.cn/page/202110/1237232.shtml
- Reuters. (2024, January 18). Dutch to use Europe’s AI Act “immediately,” invest $222 mln in sector. Reuters. https://www.reuters.com/technology/dutch-use-europes-ai-act-immediately-invest-222-mln-sector-2024-01-18/
- Schwarz, E. (2024, April 12). Gaza war: Israel using AI to identify human targets raising fears that innocents are being caught in the net. The Conversation. https://theconversation.com/gaza-war-israel-using-ai-to-identify-human-targets-raising-fears-that-innocents-are-being-caught-in-the-net-227422
- Skitka, L. (2011). Automation Bias | Linda J. Skitka, Ph.D. https://lskitka.people.uic.edu/. Retrieved September 1, 2024, from https://lskitka.people.uic.edu/styled-7/styled-14/#:~:text=Automation%20bias%20refers%20to%20a,to%20monitor%20on%2Dgoing%20tasks.
- Streitfeld, D. (2024, May 30). If AI can do your job, maybe it can also replace your CEO. The Business Times. https://www.businesstimes.com.sg/working-life/if-ai-can-do-your-job-maybe-it-can-also-replace-your-ceo
- Technica, A. B. A. (2024, February 17). Air Canada has to honor a refund policy its chatbot made up. WIRED. https://www.wired.com/story/air-canada-chatbot-refund-policy/
- Ulfert-Blank, A. S., Georganta, E., Tielman, M., & Oron-Gilad, T. (2023, January). Piecing Together the Puzzle: Understanding Trust in Human-AI Teams. In CEUR Workshop Proceedings (Vol. 3456, pp. 169-174). CEUR-WS. https://ceur-ws.org/Vol-3456/
- Vlasceanu, M., & Amodio, D. M. (2022). Propagation of societal gender inequality by internet search algorithms. Proceedings of the National Academy of Sciences, 119(29),e2204529119. https://doi.org/10.1073/pnas.2204529119
- Walsh, D. (2022, May 26). Timnit Gebru: Ethical AI requires institutional and structural change. Stanford HAI. https://hai.stanford.edu/news/timnit-gebru-ethical-ai-requires-institutional-and-structural-change