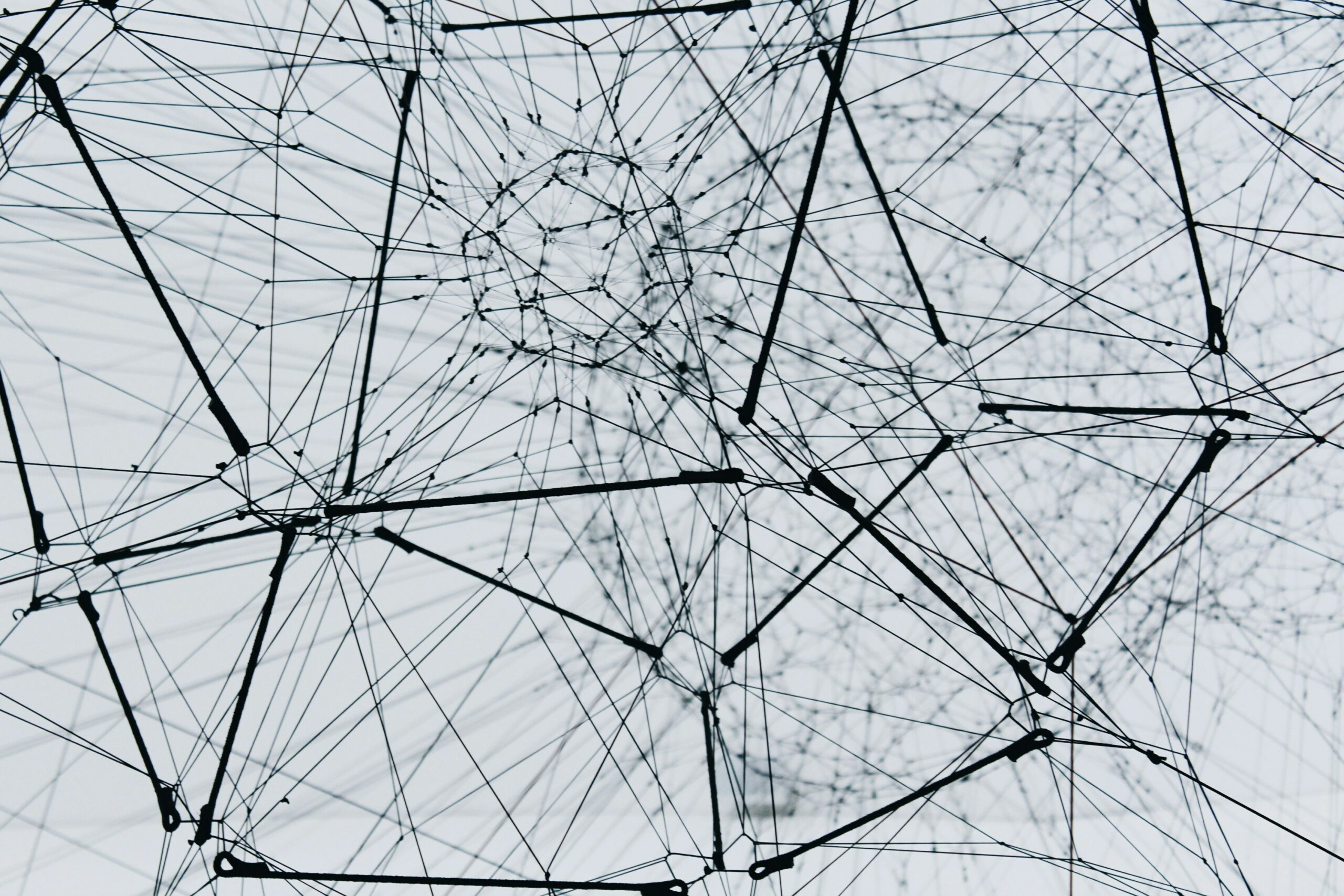
“It’s complicated, experts say” is how the Global News described the state of the COVID-19 pandemic (Wright, 2022). The more I learn, the more it seems that this is also the Answer to the Ultimate Question of Life, the Universe, and Everything.
“It’s complicated, experts say” is how the Global News described the state of the COVID-19 pandemic (Wright, 2022). The more I learn, the more it seems that this is also the Answer to the Ultimate Question of Life, the Universe, and Everything.
Photo by Alina Grubnyak
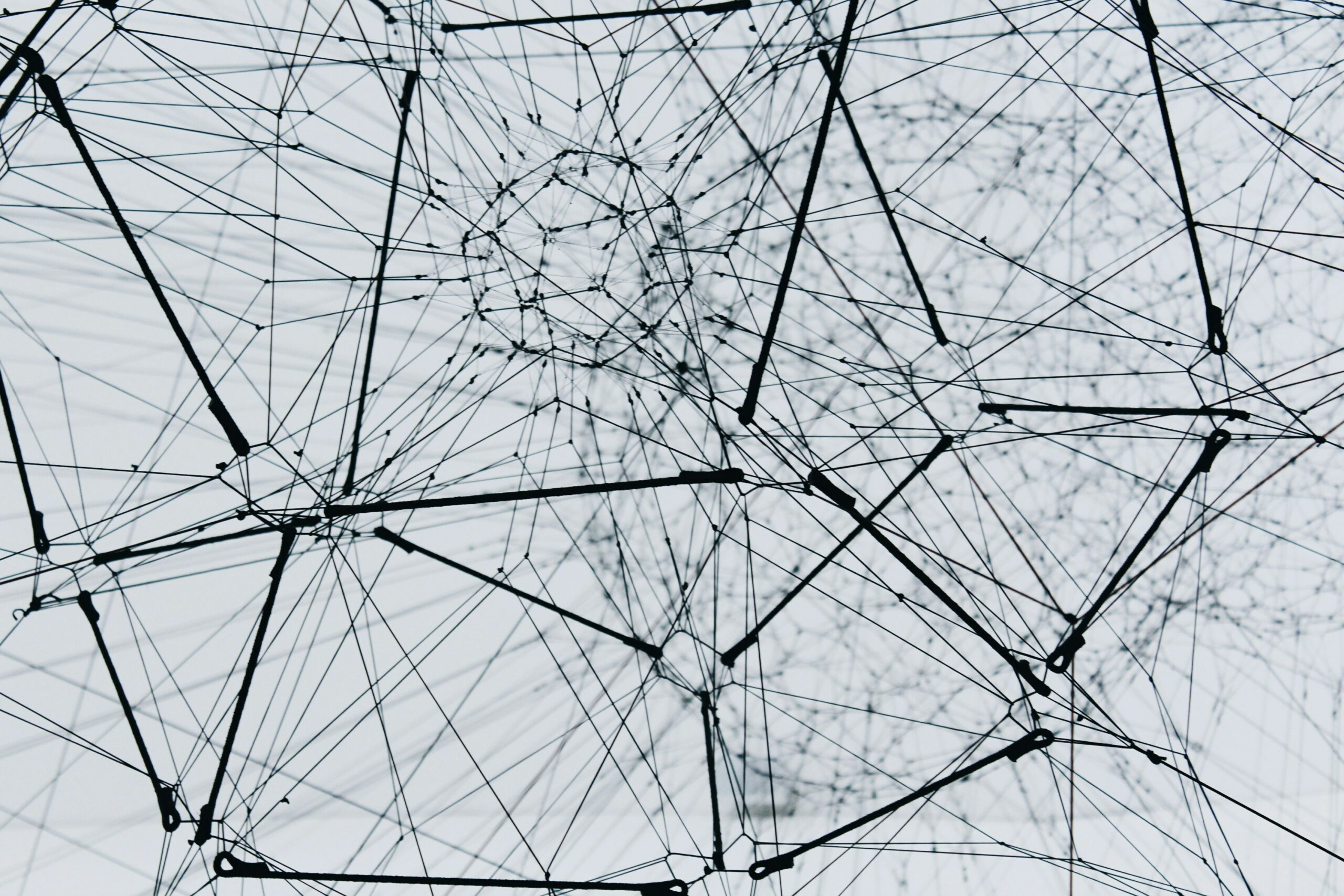
Photo by Alina Grubnyak
Try answering any of the following newspaper headlines from 2023: “How do you serve a friend in despair?” (The New York Times, 2023), “Silicon or Carbon?” (The Point Magazine, 2023), and “Can Anyone Trust The Witch Trials of J.K. Rowling?” (Vulture, 2023). When trying to understand the world, complexity is an issue for two reasons. First, humans are not that smart. We lack computing power. We can only keep “seven, plus or minus two” (Miller, 1956) things at once in mind. In a world driven by innumerable complex interconnections, how can we grasp the big picture, let alone change it for the better? The second issue lies in how scientists conduct research. The Royal Netherlands Academy of Arts and Sciences (KNAW; 2023) summarised it well: “Many information systems already exist. What is missing is the ability to integrate information from different systems” (Kort et al., 2023). Scientific literature is fragmented into individual papers, most exploring only pairs of variables, whereas the real world involves countless interacting factors. We play endless questions with nature, and then spend sleepless nights on Google Scholar trying to piece together just the tiniest fraction of the answers that we managed to uncover.
Take for example the issue pointed out by the KNAW in 2023; we are lacking an integrated framework of planetary health (an approach to health that is based on the notion that human wellbeing is dependent on the wellbeing of the planet). To make sense of planetary health, policymakers need an understanding of key factors and their interconnections, including “weather/climate, atmosphere, land use and crop yields, biodiversity, and the health and well-being of populations” (Kort et al., 2023). Try to imagine the behaviour of such a system; how a warm winter affects agricultural cycles, impacting food production and public health, and how public health might then in turn influence agricultural cycles, which have an effect on the warmth of our winter via climate change… and so forth. Can you? The bottom line; it is not enough to understand the connections between individual pairs of variables. Policymakers who make decisions about complex systems need to be aware of the dynamics that emerge when these systems’ variables interact. There is a lot going on – combine this with the fact that humans lack computing power (and time to read studies), and we are faced with a rather unfortunate set of circumstances.
In what follows, I propose (dream up) a single solution to both of these issues – Conceptualized as a network. By representing variables as nodes, and associations as weights, researchers could share a platform that keeps track of all of these variables. They could let computers do the computing, while continuously integrating and visualising global research in one unified network of associations.
“Scientific literature is fragmented into individual papers, most exploring only pairs of variables, whereas the real world involves countless interacting factors.”
From Mental Disorders to Planetary Health
In the psychology department of the UvA, the inspiration behind this idea was likely clear within my first four sentences. Denny Borsboom, in his 2017 paper A Network Theory of Mental Disorders, conceptualised mental disorders as networks of symptoms. In these networks, “symptoms are nodes, and causal interactions between symptoms are connections between nodes”. A few of the mechanisms that have been discovered within such networks include feedback loops, critical slowing down, and hysteresis (Borsboom 2017). Simply put, this tool allows researchers not only to visualise their knowledge about the interconnections of symptoms, but also to investigate the complex dynamics that emerge in the system as a whole. Recalling the problem reported by the KNAW, such networks are just what research is lacking. Networks integrate information about pairs of variables. Thus, just as nodes in a network can be “sadness” and “sleeplessness”, they can also represent “temperature”, “humidity”, and “life expectancy”. Also fun to know: Borsboom himself first heard of hysteresis in the context of ecology (Zautra, 2019). So in a way, hysteresis would come full circle if it were to be applied to planetary health by methodologists from the field of psychology.
The Network Two-Step
We are presented with a world of research on individual pairs of variables. Now additionally, we have concepts allowing us to integrate pairs of variables. Putting two and two together, the KNAW’s silver bullet might be a network of findings on planetary health. Nodes would represent variables studied in planetary health, and edges would be the relationships found between them. The creation of this network could be achieved in two steps. First, identifying the variables studied in previous research. And second, integrating the causal relationships into the network. Before the variables studied in previous papers can be identified, though, one needs to know which papers to consider. Sifting through all the available literature seems daunting, but there is hope. In a 2019 paper by Dworkin et al., the authors automated the construction of a topic network that included thousands of articles, using keywords and abstracts to identify clusters of related studies. With this approach, the vast amount of available literature can be narrowed down to a topic-specific cluster, making the workload more manageable.
“It is all the available data, in one place. Important factors can be visualised as larger nodes, to show policymakers where to focus interventions.”
Let The Minions Handle It
Yet even after reducing the amount of literature that needs to be considered, planetary health remains a vast field. If research papers are well-written, identifying the studied variables and the strength of the findings is an easy task. Doing this for all relevant papers on planetary health that were published over the past century, however, is barely feasible. Fortunately, we won’t have to do it ourselves. Over the past few years, a completely automatic way to extract relevant information from texts and store it in convenient formats for big data analysis has emerged. The ones scanning all these papers would not be armies of un(der)paid interns and undergraduate students. It would be ChatGPT, or one of its cousins (Polak & Morgan, 2024). The relevant technology is already being developed. Mutinda et al. (2022) used a “BERT-based named entity recognition (NER) model” (meaning: AI) to automate the extraction of research data from abstracts. Already in 2022, AI systems were able to extract data about participants, interventions, and outcomes from articles with around 80% accuracy. It is therefore realistic to assume that soon, the extraction of data from articles as a fully automated process can become common practice.
Grand Finale
Finally, the stage is set for policymaker-christmas. The data has now been transformed into nodes and weights. All that is left to do is to package it nicely, put it on a dashboard, and hand it to decision-makers. A visual network of variables presents an overview of the most important factors in planetary health and the interactions between them. It is all the available data, in one place. Important factors can be visualised as larger nodes, to show policymakers where to focus interventions. Data scientists could use the networks for simulations and inform policymakers about possible leverage points, vulnerabilities and dynamics like hysteresis and feedback loops (see Meadows, 2008). All these simulations, too, could be visualised and thus made intuitive. Furthermore, a network like this does not yellow with age. Whereas hundreds
of carefully written pages of meta-analyses become useless as time passes and scientists inevitably realise they were wrong, this network presents a dynamic platform. New knowledge can be integrated in real-time, and regular updates can keep the network relevant.
The Future of Data Integration?
The first person who ever heard this idea told me that they liked it because it felt like reading science fiction. Considering that automated selection as well as integration of articles are about to be a reality, I argue that these networks can be part of science, and not fiction. They offer a solution to one of the greatest problems in planetary health research, and arguably in research as a whole – bits of data, spread across countless individual journals and articles. Networks can integrate isolated findings into an intuitive, visual system that allows policymakers to opt for a data-driven focus on key variables. Through continuous updates, they present a living platform for real-time collaboration across disciplines. The potential impact is a fundamental change in the way we use research. This way of integrating data has the potential to finally move scientists in all disciplines away from a focus on individual pairs of variables, and instead one step closer to seeing the systems of our world as they are – complex.
References
- Borsboom, D. (2017). A network theory of mental disorders. World Psychiatry, 16(1), 5–13. https://doi.org/10.1002/wps.20375
- Dworkin, J. D., Shinohara, R. T., & Bassett, D. S. (2019). The emergent integrated network structure of scientific research. PLoS ONE, 14(4), e0216146. https://doi.org/10.1371/journal.pone.0216146
- Interaction Design Foundation. (2024, September 27). How to Display Complex Network Data with Information Visualization. The Interaction Design Foundation. https://www.interaction-design.org/literature/article/how-to-display-complex-network-data-with-information-visualization
- Kort, R., Arts, K., Antó, J. M., Berg, M. P., Cepella, G., Cole, J., Van Doorn, A., Van Gorp, T., Grootjen, M., Gupta, J., Hill, C., Van Der Heide, E., Huisman, J., Janmaat, J., O’Callaghan-Gordo, C., Mattijsen, J., Modi, T., Nowak, E., Ossebaard, H. C., . . . Martens, P. (2023). Outcomes from the First European Planetary Health Congress at ARTIS in Amsterdam. Challenges, 14(4), 49. https://doi.org/10.3390/challe14040049
- Meadows, D. H. (2008). Thinking in systems: A Primer. Chelsea Green Publishing.
- Miller, G. A. (1956). The magical number seven, plus or minus two: Some limits on our capacity for processing information. Psychological Review, 63(2), 81–97. https://doi.org/10.1037/h0043158
- Mutinda, F. W., Liew, K., Yada, S., Wakamiya, S., & Aramaki, E. (2022). Automatic data extraction to support meta-analysis statistical analysis: a case study on breast cancer. BMC Medical Informatics and Decision Making, 22(1). https://doi.org/10.1186/s12911-022-01897-4
- Polak, M. P., & Morgan, D. (2024). Extracting accurate materials data from research papers with conversational language models and prompt engineering. Nature Communications, 15(1). https://doi.org/10.1038/s41467-024-45914-8
- The New York Times. (2023, December 27). The most popular Times articles of 2023. The New York Times. https://www.nytimes.com/2023/12/26/briefing/most-read-times-journalism.html
- The Point Magazine. (2023, December 28). Top articles of 2023. https://thepointmag.com/general/top-articles-of-2023/
- Vulture. (2023, December 25). Vulture’s Most-Read Stories of 2023: The Year In Review. Vulture. https://www.vulture.com/article/most-read-stories-2023.html
- Wright, T. (2022, September 22). When will the COVID-19 pandemic officially be over? It’s complicated, experts say. Global News. https://globalnews.ca/news/9149120/covid-pandemic-over-complicated-experts/
- Zautra, N., Borsboom, D. (2019, April). Denny Borsboom (No. 62) [Audio podcast episode]. SCI PHI Podcast. Spotify., https://open.spotify.com/episode/1yVTZmMNB8CtItjnQXrh1W?si=396a4d4fa34d4fe1.
Try answering any of the following newspaper headlines from 2023: “How do you serve a friend in despair?” (The New York Times, 2023), “Silicon or Carbon?” (The Point Magazine, 2023), and “Can Anyone Trust The Witch Trials of J.K. Rowling?” (Vulture, 2023). When trying to understand the world, complexity is an issue for two reasons. First, humans are not that smart. We lack computing power. We can only keep “seven, plus or minus two” (Miller, 1956) things at once in mind. In a world driven by innumerable complex interconnections, how can we grasp the big picture, let alone change it for the better? The second issue lies in how scientists conduct research. The Royal Netherlands Academy of Arts and Sciences (KNAW; 2023) summarised it well: “Many information systems already exist. What is missing is the ability to integrate information from different systems” (Kort et al., 2023). Scientific literature is fragmented into individual papers, most exploring only pairs of variables, whereas the real world involves countless interacting factors. We play endless questions with nature, and then spend sleepless nights on Google Scholar trying to piece together just the tiniest fraction of the answers that we managed to uncover.
Take for example the issue pointed out by the KNAW in 2023; we are lacking an integrated framework of planetary health (an approach to health that is based on the notion that human wellbeing is dependent on the wellbeing of the planet). To make sense of planetary health, policymakers need an understanding of key factors and their interconnections, including “weather/climate, atmosphere, land use and crop yields, biodiversity, and the health and well-being of populations” (Kort et al., 2023). Try to imagine the behaviour of such a system; how a warm winter affects agricultural cycles, impacting food production and public health, and how public health might then in turn influence agricultural cycles, which have an effect on the warmth of our winter via climate change… and so forth. Can you? The bottom line; it is not enough to understand the connections between individual pairs of variables. Policymakers who make decisions about complex systems need to be aware of the dynamics that emerge when these systems’ variables interact. There is a lot going on – combine this with the fact that humans lack computing power (and time to read studies), and we are faced with a rather unfortunate set of circumstances.
In what follows, I propose (dream up) a single solution to both of these issues – Conceptualized as a network. By representing variables as nodes, and associations as weights, researchers could share a platform that keeps track of all of these variables. They could let computers do the computing, while continuously integrating and visualising global research in one unified network of associations.
“Scientific literature is fragmented into individual papers, most exploring only pairs of variables, whereas the real world involves countless interacting factors.”
From Mental Disorders to Planetary Health
In the psychology department of the UvA, the inspiration behind this idea was likely clear within my first four sentences. Denny Borsboom, in his 2017 paper A Network Theory of Mental Disorders, conceptualised mental disorders as networks of symptoms. In these networks, “symptoms are nodes, and causal interactions between symptoms are connections between nodes”. A few of the mechanisms that have been discovered within such networks include feedback loops, critical slowing down, and hysteresis (Borsboom 2017). Simply put, this tool allows researchers not only to visualise their knowledge about the interconnections of symptoms, but also to investigate the complex dynamics that emerge in the system as a whole. Recalling the problem reported by the KNAW, such networks are just what research is lacking. Networks integrate information about pairs of variables. Thus, just as nodes in a network can be “sadness” and “sleeplessness”, they can also represent “temperature”, “humidity”, and “life expectancy”. Also fun to know: Borsboom himself first heard of hysteresis in the context of ecology (Zautra, 2019). So in a way, hysteresis would come full circle if it were to be applied to planetary health by methodologists from the field of psychology.
The Network Two-Step
We are presented with a world of research on individual pairs of variables. Now additionally, we have concepts allowing us to integrate pairs of variables. Putting two and two together, the KNAW’s silver bullet might be a network of findings on planetary health. Nodes would represent variables studied in planetary health, and edges would be the relationships found between them. The creation of this network could be achieved in two steps. First, identifying the variables studied in previous research. And second, integrating the causal relationships into the network. Before the variables studied in previous papers can be identified, though, one needs to know which papers to consider. Sifting through all the available literature seems daunting, but there is hope. In a 2019 paper by Dworkin et al., the authors automated the construction of a topic network that included thousands of articles, using keywords and abstracts to identify clusters of related studies. With this approach, the vast amount of available literature can be narrowed down to a topic-specific cluster, making the workload more manageable.
“It is all the available data, in one place. Important factors can be visualised as larger nodes, to show policymakers where to focus interventions.”
Let The Minions Handle It
Yet even after reducing the amount of literature that needs to be considered, planetary health remains a vast field. If research papers are well-written, identifying the studied variables and the strength of the findings is an easy task. Doing this for all relevant papers on planetary health that were published over the past century, however, is barely feasible. Fortunately, we won’t have to do it ourselves. Over the past few years, a completely automatic way to extract relevant information from texts and store it in convenient formats for big data analysis has emerged. The ones scanning all these papers would not be armies of un(der)paid interns and undergraduate students. It would be ChatGPT, or one of its cousins (Polak & Morgan, 2024). The relevant technology is already being developed. Mutinda et al. (2022) used a “BERT-based named entity recognition (NER) model” (meaning: AI) to automate the extraction of research data from abstracts. Already in 2022, AI systems were able to extract data about participants, interventions, and outcomes from articles with around 80% accuracy. It is therefore realistic to assume that soon, the extraction of data from articles as a fully automated process can become common practice.
Grand Finale
Finally, the stage is set for policymaker-christmas. The data has now been transformed into nodes and weights. All that is left to do is to package it nicely, put it on a dashboard, and hand it to decision-makers. A visual network of variables presents an overview of the most important factors in planetary health and the interactions between them. It is all the available data, in one place. Important factors can be visualised as larger nodes, to show policymakers where to focus interventions. Data scientists could use the networks for simulations and inform policymakers about possible leverage points, vulnerabilities and dynamics like hysteresis and feedback loops (see Meadows, 2008). All these simulations, too, could be visualised and thus made intuitive. Furthermore, a network like this does not yellow with age. Whereas hundreds
of carefully written pages of meta-analyses become useless as time passes and scientists inevitably realise they were wrong, this network presents a dynamic platform. New knowledge can be integrated in real-time, and regular updates can keep the network relevant.
The Future of Data Integration?
The first person who ever heard this idea told me that they liked it because it felt like reading science fiction. Considering that automated selection as well as integration of articles are about to be a reality, I argue that these networks can be part of science, and not fiction. They offer a solution to one of the greatest problems in planetary health research, and arguably in research as a whole – bits of data, spread across countless individual journals and articles. Networks can integrate isolated findings into an intuitive, visual system that allows policymakers to opt for a data-driven focus on key variables. Through continuous updates, they present a living platform for real-time collaboration across disciplines. The potential impact is a fundamental change in the way we use research. This way of integrating data has the potential to finally move scientists in all disciplines away from a focus on individual pairs of variables, and instead one step closer to seeing the systems of our world as they are – complex.
References
- Borsboom, D. (2017). A network theory of mental disorders. World Psychiatry, 16(1), 5–13. https://doi.org/10.1002/wps.20375
- Dworkin, J. D., Shinohara, R. T., & Bassett, D. S. (2019). The emergent integrated network structure of scientific research. PLoS ONE, 14(4), e0216146. https://doi.org/10.1371/journal.pone.0216146
- Interaction Design Foundation. (2024, September 27). How to Display Complex Network Data with Information Visualization. The Interaction Design Foundation. https://www.interaction-design.org/literature/article/how-to-display-complex-network-data-with-information-visualization
- Kort, R., Arts, K., Antó, J. M., Berg, M. P., Cepella, G., Cole, J., Van Doorn, A., Van Gorp, T., Grootjen, M., Gupta, J., Hill, C., Van Der Heide, E., Huisman, J., Janmaat, J., O’Callaghan-Gordo, C., Mattijsen, J., Modi, T., Nowak, E., Ossebaard, H. C., . . . Martens, P. (2023). Outcomes from the First European Planetary Health Congress at ARTIS in Amsterdam. Challenges, 14(4), 49. https://doi.org/10.3390/challe14040049
- Meadows, D. H. (2008). Thinking in systems: A Primer. Chelsea Green Publishing.
- Miller, G. A. (1956). The magical number seven, plus or minus two: Some limits on our capacity for processing information. Psychological Review, 63(2), 81–97. https://doi.org/10.1037/h0043158
- Mutinda, F. W., Liew, K., Yada, S., Wakamiya, S., & Aramaki, E. (2022). Automatic data extraction to support meta-analysis statistical analysis: a case study on breast cancer. BMC Medical Informatics and Decision Making, 22(1). https://doi.org/10.1186/s12911-022-01897-4
- Polak, M. P., & Morgan, D. (2024). Extracting accurate materials data from research papers with conversational language models and prompt engineering. Nature Communications, 15(1). https://doi.org/10.1038/s41467-024-45914-8
- The New York Times. (2023, December 27). The most popular Times articles of 2023. The New York Times. https://www.nytimes.com/2023/12/26/briefing/most-read-times-journalism.html
- The Point Magazine. (2023, December 28). Top articles of 2023. https://thepointmag.com/general/top-articles-of-2023/
- Vulture. (2023, December 25). Vulture’s Most-Read Stories of 2023: The Year In Review. Vulture. https://www.vulture.com/article/most-read-stories-2023.html
- Wright, T. (2022, September 22). When will the COVID-19 pandemic officially be over? It’s complicated, experts say. Global News. https://globalnews.ca/news/9149120/covid-pandemic-over-complicated-experts/
- Zautra, N., Borsboom, D. (2019, April). Denny Borsboom (No. 62) [Audio podcast episode]. SCI PHI Podcast. Spotify., https://open.spotify.com/episode/1yVTZmMNB8CtItjnQXrh1W?si=396a4d4fa34d4fe1.