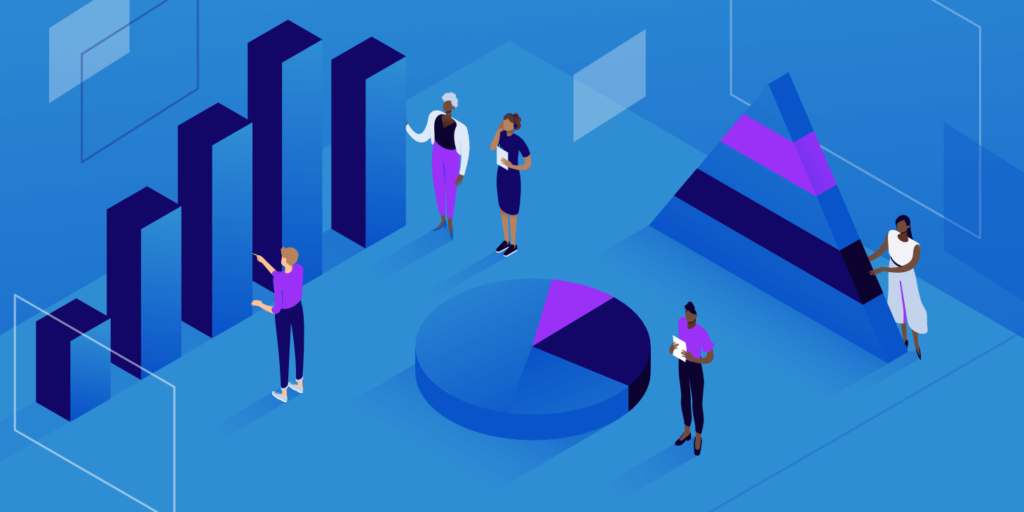
The term ‘mathematical psychology’ might to some of you sound like an oxymoron, a phrase that contains two irreconcilable words – mathematics and psychology. However, mathematical psychology is an actual discipline in psychology that is defined as “an approach to psychological phenomena that uses mathematical techniques to model the underlying processes and to predict the outcomes of these processes.” (APA, n.d.) Mathematical psychology is thus clearly related to psychometrics and statistics, the first being concerned mainly with the quantification of psychological constructs, improvement of measurement instruments, and research methodology, and the latter being concerned mainly with, well, the mathematics of such quantification. In this way, both psychometrics and statistics could be viewed as the how of psychology – how the research is conducted – while mathematical psychology could be seen as the what, or content of certain subdisciplines in psychology, mainly cognitive psychology, psychophysics, and perception.
Even though mathematical psychology might sound repulsive to most psychology students, this branch of psychology has great value. First, by modeling psychological processes, researchers must be explicit about their assumptions with respect to the effect of one variable on another as they are modeling the behaviour mathematically. This precision brings us all closer to actually understanding how X influences Y. For example, the relationship between X and Y could be linear, or sigmoid, or totally different. The maths in mathematical psychology forces us to specify. Second, modeling psychological processes makes our predictions about the phenomena more precise, and therefore more testable and falsifiable. This precision that mathematical psychology offers is just some good science. Below are some resources you can use to delve a little deeper into the topic of mathematical psychology.
Thanks to its focus on quantitative rather than qualitative research methods, the Department of Psychological Methods at our University of Amsterdam is quite prolific and well-known for contributing to the field of psychometrics and research methods, featuring quite popular researchers such as Adriaan de Groot, Don Mellenbergh, Han van der Maas, Eric-Jan Wagenmakers, and Denny Borsboom, among many many other innovative researchers and PhD students. This is why many of the resources below could be directly tied to our Department of Psychological Methods.
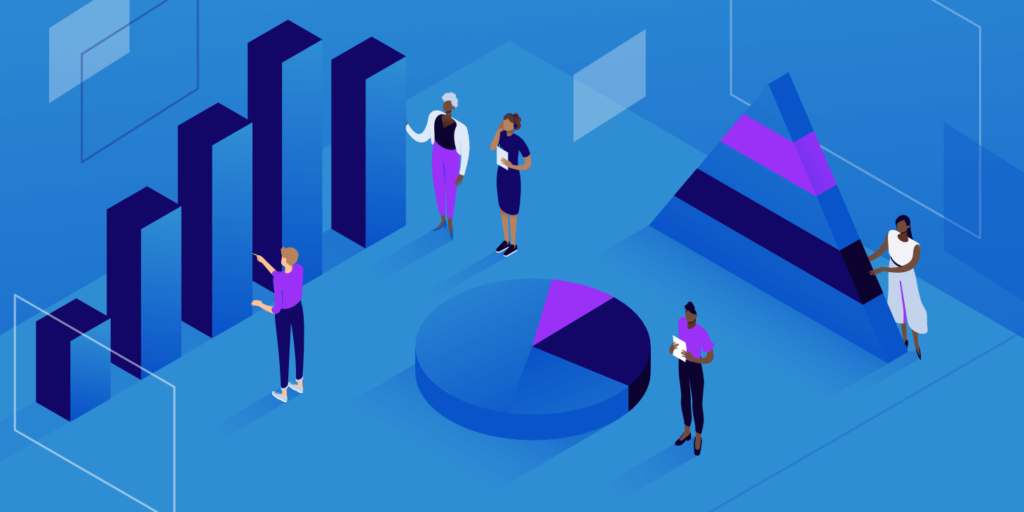
- Eric-Jan Wagenmakers: Bayesian Thinking for Toddlers
Bayesian thinking and the related Bayesian statistics is an alternative to the mainstream, status-quo frequentist statistics. It is up to you to decide whether a toddler would understand the book, but one thing is certain: Eric-Jan guides you through the main principles and premises of Bayesian thinking with down-to-earth examples, featuring princesses and dragons. It is definitely an enjoyable read.
- Nate Silver: The Signal and the Noise
Nate Silver, the famous guy who correctly predicted the outcome of the US presidential election in 2016, discusses how to make good predictions. As a statistician, he discusses a range of topics from weather to basketball to earthquakes, touching upon intriguing concepts such as complex dynamical systems and chaos theory – and how we humans can be so easily tricked into believing what we see is the signal in the data, while we’re fooled by the noise.
- Denny Borsboom: Measuring the Mind
Denny discusses the conceptual issues in contemporary psychometrics, writing about topics such as classical test theory and latent variable models. Denny is taking three perspectives: formal/logical, empirical, and ontological. If you’re looking for a comprehensive and well-argued read on the topic of psychological measurement, definitely go for this book.
Sometimes it’s more comfortable to just sit back and watch a video. Instead of watching cat videos, though, there are some Youtube channels witch may spark your interest in mathematical psychology.
This channel offers a variety of data science explanations, using funny and practical examples, such as explaining how police are using calculus to find out whether you’re speeding. You’ll also find loads of videos explaining seemingly difficult concepts such as random forests or the tricky eigenvalues and eigenvectors.
We can’t omit this famous channel when talking about mathematical psychology. This channel offers beautiful visualisations of even the most abstract concepts such as calculus or neural networks. These are very relevant for mathematical psychology as they are the starting points from which one goes on and builds a statistical model of some psychological phenomena.
- Machine Learning by the Stanford University
Stanford University offers a course by Andrew Ng called Machine learning, entirely on YouTube. As intimidating as it sounds, this course is quite informative about statistical concepts such as bias and variance, or overfitting and underfitting, all topics that are very relevant to mathematical psychology. If you’re short on free time, you can also watch the lectures separately – they do make sense even in different order.
This course is offered as part of the psychological methods specialisation, but generally you can take it as a second or third year psychology student. It is more conceptual than technical, and provides a smooth transition from formulas such as the Bayes rule to the actual reasoning behind those formulas, and thus attempts to close the gap between Bayesian Statistics and Bayesian Framework.
This course is offered by the Institute of Interdisciplinary studies of the UvA and can be a fascinating element of your regular bachelor or master degree (you can also take it if you’re not a student). Topics covered include defining and understanding complex systems, and how to visualise and simulate them yourself.
In honours of the previous programme leader at the Psychological Methods department at the UvA, Don Mellenbergh, members of the Psychological Methods student faction of the UvA organise monthly lectures on any topic related to psychological methods, such as neural networks or infant cognition, by experts in the field from all around the world, including Stanford, Groningen, Tilburg and Amsterdam. Recordings of lectures are posted later on this YouTube channel. A typical lecture consists of a 40-minute presentation by the researcher and a 15-minute discussion with the attendees. Additionally, you can get a colloquium point for attending these lectures.
There are countless articles that I would like to include as part of this column. However, for practical purposes, I can mention only a few:
No, this is not a paper by Dylan. This article argues why knowledge about the group (or interindividual variability) might not be informative about the individual (intraindividual variability), and suggests that if and only if certain conditions are met (a condition called ergodicity), these two are informative about each other. A great conceptual read to understand why psychological processes on the aggregate might not shed light on an individual.
As a fresh way to look at mental disorders, Denny proposes a model where depression can be seen as a state of a self-reinforcing network of symptoms, persistent even after the external cause is removed. This paper beautifully illustrates how mathematical psychology and network analysis can inform other branches of psychology, such as clinical psychology.
Mathematical psychology is a very hands-on branch of psychology, and you might need some statistical and programming skills to delve deeper into it.
Generally speaking, R is an intuitive language for mathematical simulation and modelling. In this lecture, you can learn how to create your own app (using R, in what is called R Shiny) in order to collect data as a research study which you can later analyse. However, Python might be used as well.
Once you have collected the data you wish to analyse, it is convenient to use open-source software (which means it’s free!) such as JASP which has been developed by researchers at the UvA. JASP also has a friendly user interface and is constantly improving, continuously adding new useful features such as the machine learning module. Above all, JASP is your best friend when it comes to analysing data using Bayesian statistics, since it produces an easily readable and labelled output.